-
摘要: 脱机签名验证模型因其判断签名是否伪造的能力而备受关注. 当今大多数脱机签名验证模型可分为深度度量学习方法和双通道判别方法. 大部分深度度量学习方法利用孪生网络生成每张图片的细节特征向量, 采用欧氏距离法判断相似度, 但是欧氏距离仅考虑两个点之间的绝对距离, 而容易忽视点的方向、缩放的信息, 不会考虑数据之间的相关性, 因此无法捕获特征向量内部之间的关系; 而双通道判别方法在网络前就进行特征的判别, 更能判断不同图像的相似性, 但此时图像的细节特征不够清晰, 大量特征丢失. 针对双通道判别方法中特征消失过多的问题, 提出了一种面向独立于书写者场景的手写签名离线验证模型(Multi-channel feature fusion network, MCFFN). MCFFN 模型首先通过多重网络和双重逆鉴别注意力模块进行特征提取, 然后通过多通道机制进行特征融合, 最后使用 ACMix 判别模块获得预测结果. 在 CEDAR、BHSig-B、BHSig-H 和 ChiSig 四个不同语言的签名数据集上测试了所提出的方法, 实验证明了所提方法的优势和潜力.Abstract: The offline signature verification model has garnered considerable attention due to its ability to discern the authenticity of signatures. Presently, most offline signature verification models can be categorized into deep metric learning approaches and 2-channel discriminative methods. Most of deep metric learning methods use Siamese network to generate detailed feature vectors for each image, and the Euclidean distance method is used to determine the similarity, However, the Euclidean distance only considers the absolute distance between two points, and it is easy to overlook the direction and scaling information of points. The correlation between data will not be considered, so unable to capture relationships within feature vectors. On the other hand, 2-channel discriminative methods perform feature discrimination before the model training, enhancing the ability to determine the dissimilarity between different images. However, in this case, the fine details of the images are not sufficiently clear, resulting in a significant loss of features. Addressing the issue of excessive feature loss in 2-channel discriminative methods, this paper introduces a handwritten signature offline verification model designed for scenarios independent of the writer (Multi-channel feature fusion network, MCFFN). The MCFFN model initiates feature extraction through multiple networks and dual reverse discriminative attention modules. Subsequently, it employs multi-channel fusion for feature integration, culminating in prediction outcomes using the ACMix discriminative module. The efficacy and potential of the proposed method were validated through experiments conducted on four distinct language signature datasets: CEDAR, BHSig-B, BHSig-H, and ChiSig. The experimental results affirm the advantages and potential of the proposed approach.
-
表 1 脱机签名验证数据集
Table 1 Offline signature verification dataset
数据集名称 语言 签名种类 图片数量 真实伪造样本比 CEDAR 英语 55 2624 24/24 BHSig260-B 孟加拉语 100 5400 24/30 BHSig260-H 印地语 160 8640 24/30 ChiSig 中文 102 10242 −/− 表 2 基于CEDAR数据集的对比实验
Table 2 Comparison on CEDAR dataset
模型名称 FRR FAR ACC SigNet (2017arXiv) 0 0 100 DeepHSV (2019ICDAR) — — 100 IDN (2019CVPR) 2.17 5.87 96.38 SDINet (2021AAAI) 3.42 0.73 98.25 2C2S (2023EAAI) 0 0 100 OURS 0 0 100 表 3 基于BHSig-B数据集的对比实验
Table 3 Comparison on BHSig-B dataset
模型名称 FRR FAR ACC SigNet (2017arXiv) 13.89 13.89 86.11 DeepHSV (2019ICDAR) — — 88.08 IDN (2019CVPR) 5.24 4.12 95.32 SDINet (2021AAAI) 7.86 3.30 94.42 SURDS (2022ICPR) 5.42 19.89 87.34 2C2S (2023EAAI) 8.11 5.37 93.25 TransOSV (2022ICME) 9.95 9.95 90.05 OURS 3.86 3.84 95.61 表 4 基于BHSig-H数据集的对比实验
Table 4 Comparison on BHSig-H dataset
模型名称 FRR FAR ACC SigNet (2017arXiv) 15.36 15.36 84.64 DeepHSV (2019ICDAR) — — 86.66 IDN (2019CVPR) 4.93 8.99 93.04 SDINet (2021AAAI) 3.77 6.24 95.00 SURDS (2022ICPR) 8.98 12.01 89.50 2C2S (2023EAAI) 9.98 8.66 90.68 TransOSV (2022ICME) 3.39 3.39 96.61 OURS 4.89 4.89 95.7 表 5 基于ChiSig数据集的消融实验
Table 5 Ablation experiment on ChiSig dataset
模型名称 EER TAR ACC InceptionResnet 6.6 28.1 93.6 SigNet — — 82.28 IDN (基线) 17.91 10.5 84.82 IDN (通道融合) 14.81 9.61 85.72 IDN (通道融合+注意力) 11.38 7.82 88.96 OURS (无反灰度图片, 无注意力) 11.78 32.49 88.09 OURS (无反灰度图片, 单注意力) 10.83 — 89.20 OURS (反灰度图片, 无注意力) 7.84 — 92.14 OURS 5.19 28.96 95.23 表 6 基于ChiSig数据集的主流参数
Table 6 Main parameter on ChiSig dataset
模型名称 FRR FAR ACC IDN 10.46 17.91 84.82 IDN (通道融合) 9.61 18.97 85.72 IDN (通道融合+注意力) 7.82 14.27 88.96 OURS (无反灰度图片, 无注意力) 21.91 17.26 88.09 OURS (无反灰度图片, 单注意力) 15.59 16.30 89.20 OURS (反灰度图片, 无注意力) 6.90 17.18 92.14 OURS 5.34 5.34 95.23 表 7 跨语言实验
Table 7 Cross-language test
训练集/测试集 CEDAR BHSig-B BHSig-H ChiSig CEDAR 100 48.76 49.89 57.48 BHSig-B 64.86 95.61 82.79 63.71 BHSig-H 50.11 86.27 95.7 20 ChiSig 54.60 70.02 55.37 95.23 -
[1] Bromley J, Bentz J W, Bottou L, Guyon I, Lecun Y, Moore C, et al . Signature verification using a “Siamese” time delay neural network. International Journal of Pattern Recognition and Artificial Intelligence,1993 ,7 (4 ):669 −688 doi: 10.1142/S0218001493000339[2] Cpałka K, Zalasiński M, Rutkowski L . New method for the on-line signature verification based on horizontal partitioning. Pattern Recognition,2014 ,47 (8 ):2652 −2661 doi: 10.1016/j.patcog.2014.02.012[3] Xia X H, Song X Y, Luan F G, Zheng J G, Chen Z L, Ma X F . Discriminative feature selection for on-line signature verification. Pattern Recognition,2018 ,74 :422 −433 doi: 10.1016/j.patcog.2017.09.033[4] 邹杰, 孙宝林, 於俊 . 基于笔画特征的在线笔迹匹配算法. 自动化学报,2016 ,42 (11 ):1744 −1757 Zou Jie, Sun Bao-Lin, Yu Jun . Online handwriting matching algorithm based on stroke features. Acta Automatica Sinica,2016 ,42 (11 ):1744 −1757 [5] Guerbai Y, Chibani Y, Hadjadji B . The effective use of the one-class SVM classifier for handwritten signature verification based on writer-independent parameters. Pattern Recognition,2015 ,48 (1 ):103 −113 doi: 10.1016/j.patcog.2014.07.016[6] Hu J, Chen Y B. Offline signature verification using real adaboost classifier combination of pseudo-dynamic features. In: Proceedings of the 12th International Conference on Document Analysis and Recognition. Washington, USA: IEEE, 2013. 1345−1349 [7] Kumar R, Sharma J D, Chanda B . Writer-independent off-line signature verification using surroundedness feature. Pattern Recognition Letters,2012 ,33 (3 ):301 −308 doi: 10.1016/j.patrec.2011.10.009[8] Hafemann L G, Oliveira L S, Sabourin R . Fixed-sized representation learning from offline handwritten signatures of different sizes. International Journal on Document Analysis and Recognition (IJDAR),2018 ,21 (3 ):219 −232 doi: 10.1007/s10032-018-0301-6[9] Hafemann L G, Sabourin R, Oliveira L S . Learning features for offline handwritten signature verification using deep convolutional neural networks. Pattern Recognition,2017 ,70 :163 −176 doi: 10.1016/j.patcog.2017.05.012[10] Okawa M . Synergy of foreground-background images for feature extraction: Offline signature verification using Fisher vector with fused KAZE features. Pattern Recognition,2018 ,79 :480 −489 doi: 10.1016/j.patcog.2018.02.027[11] Xing Z J, Yin F, Wu Y C, Liu C L. Offline signature verification using convolution Siamese network. In: Proceedings of SPIE 10615, 9th International Conference on Graphic and Image Processing (ICGIP). Qingdao, China: SPIE, 2017. 415-423 [12] Soleimani A, Araabi B N, Fouladi K . Deep multitask metric learning for offline signature verification. Pattern Recognition Letters,2016 ,80 :84 −90 doi: 10.1016/j.patrec.2016.05.023[13] Jain A K, Ross A, Prabhakar S . An introduction to biometric recognition. IEEE Transactions on Circuits and Systems for Video Technology,2004 ,14 (1 ):4 −20 [14] Kalera M K, Srihari S, Xu A H . Offline signature verification and identification using distance statistics. International Journal of Pattern Recognition and Artificial Intelligence,2004 ,18 (7 ):1339 −1360 doi: 10.1142/S0218001404003630[15] Ferrer M A, Alonso J B, Travieso C M . Offline geometric parameters for automatic signature verification using fixed-point arithmetic. IEEE Transactions on Pattern Analysis And Machine Intelligence,2005 ,27 (6 ):993 −997 doi: 10.1109/TPAMI.2005.125[16] Pal S, Alaei A, Pal U, Blumenstein M. Performance of an off-line signature verification method based on texture features on a large Indic-script signature dataset. In: Proceedings of the 12th IAPR workshop on Document Analysis Systems (DAS). Santorini, Greece: IEEE, 2016. 72−77 [17] Zhang P R, Jiang J J, Liu Y L, Jin L W. MSDS: A large-Scale Chinese signature and token digit string dataset for handwriting verification. In: Proceeding of the 36th International Conference on Neural Information Processing Systems. New Orleans, USA, 2022. 36507−36519 (查阅网上资料, 未找到对应的出版者信息, 请确认) [18] Yan K H, Zhang Y, Tang H R, Ren C K, Zhang J, Wang G A, et al. Signature detection, restoration, and verification: A novel Chinese document signature forgery detection benchmark. In: Proceedings of the IEEE/CVF Conference on Computer Vision and Pattern Recognition Workshops (CVPRW). New Orleans, USA: IEEE, 2022. 5163−5172 [19] Nagel R N, Rosenfeld A. Steps toward handwritten signature verification. In: Proceedings of the 1st International Joint Conference on Pattern Recognition. 1973. 59−66 (查阅网上资料, 未找到对应的出版信息, 请确认) [20] Herbst N M, Liu C N . Automatic signature verification based on accelerometry. IBM Journal of Research and Development,1977 ,21 (3 ):245 −253 doi: 10.1147/rd.213.0245[21] 刘成林, 刘迎建, 戴汝为 . 基于多通道分解与匹配的笔迹鉴别研究. 自动化学报,1997 ,23 (1 ):56 −63 Liu Cheng-Lin, Liu Ying-Jian, Dai Ru-Wei . Writer identification by multichannel decomposition and matching. Acta Automatica Sinica,1997 ,23 (1 ):56 −63 [22] 朱勇, 谭铁牛, 王蕴红 . 基于笔迹的身份鉴别. 自动化学报,2001 ,27 (2 ):229 −234 Zhu Yong, Tan Tie-Niu, Wang Yun-Hong . Writer identification based on texture analysis. Acta Automatica Sinica,2001 ,27 (2 ):229 −234 [23] Dey S, Dutta A, Toledo J I, Ghosh S K, Llados J, Pal U. SigNet: Convolutional Siamese network for writer independent offline signature verification. arXiv preprint arXiv: 1707.02131, 2017. (查阅网上资料, 请核对文献类型及格式) [24] Li C, Lin F, Wang Z Y, Yu G, Yuan L, Wang H Q. DeepHSV: User-independent offline signature verification using two-channel CNN. In: Proceedings of the International Conference on Document Analysis and Recognition (ICDAR). Sydney, Australia: IEEE, 2019. 166−171 [25] Wei P, Li H, Hu P. Inverse discriminative networks for handwritten signature verification. In: Proceedings of the IEEE/CVF Conference on Computer Vision and Pattern Recognition (CVPR). Long Beach, USA: IEEE, 2019. 5764−5772 [26] Li H, Wei P, Ma Z Y, Li C K, Zheng N N. Offline signature verification with transformers. In: Proceedings of the IEEE International Conference on Multimedia and Expo (ICME). Taipei, China: IEEE, 2022. 1−6 [27] Cairang X M, Zhaxi D J, Yang X L, Hou Y, Zhao Q J, Gao D G, et al. Learning generalisable representations for offline signature verification. In: Proceedings of the International Joint Conference on Neural Networks (IJCNN). Padua, Italy: IEEE, 2022. 1−7 [28] Vaswani A, Shazeer N, Parmar N, Uszkoreit J, Jones L, Gomez A N, et al. Attention is all you need. In: Proceedings of the 31st International Conference on Neural Information Processing Systems. Long Beach, USA: Curran Associates Inc., 2017. 6000-6010 [29] Dosovitskiy A, Beyer L, Kolesnikov A, Weissenborn D, Zhai X H, Unterthiner T, et al. An image is worth 16x16 words: Transformers for image recognition at scale. In: Proceedings of the 9th International Conference on Learning Representations. Austria: OpenReview.net, 2021. (查阅网上资料, 未找到对应的出版城市信息, 请确认) [30] Pan X R, Ge C J, Lu R, Song S J, Chen G F, Huang Z Y, et al. On the integration of self-attention and convolution. In: Proceedings of the IEEE/CVF Conference on Computer Vision and Pattern Recognition (CVPR). New Orleans, USA: IEEE, 2022. 815−825 [31] Bromley J, Guyon I, LeCun Y, Säckinger E, Shah R. Signature verification using a “Siamese” time delay neural network. In: Proceedings of the 6th International Conference on Neural Information Processing Systems. Denver, Colorado: Morgan Kaufmann Publishers Inc., 1993. 737−744 [32] Bhattacharya I, Ghosh P, Biswas S . Offline signature verification using pixel matching technique. Procedia Technology,2013 ,10 :970 −977 doi: 10.1016/j.protcy.2013.12.445[33] Liu L, Huang L L, Yin F, Chen Y B . Offline signature verification using a region based deep metric learning network. Pattern Recognition,2021 ,118 :Article No. 108009 doi: 10.1016/j.patcog.2021.108009[34] Li H, Wei P, Hu P. Static-dynamic interaction networks for offline signature verification. In: Proceedings of 35th the AAAI Conference on Artificial Intelligence. AAAI Press, 2021. 1893-1901 (查阅网上资料, 未找到对应的出版地信息, 请确认) [35] Chattopadhyay S, Manna S, Bhattacharya S, Pal U. SURDS: Self-supervised attention-guided reconstruction and dual triplet loss for writer independent offline signature verification. In: Proceedings of the 26th International Conference on Pattern Recognition (ICPR). Montreal, Canada: IEEE, 2022. 1600−1606 [36] Zagoruyko S, Komodakis N. Learning to compare image patches via convolutional neural networks. In: Proceedings of the IEEE Conference on Computer Vision and Pattern Recognition (CVPR). Boston, USA: IEEE, 2015. 4353−4361 [37] Ren J X, Xiong Y J, Zhan H J, Huang B . 2C2S: A two-channel and two-stream transformer based framework for offline signature verification. Engineering Applications of Artificial Intelligence,2023 ,118 :Article No. 105639 doi: 10.1016/j.engappai.2022.105639 -
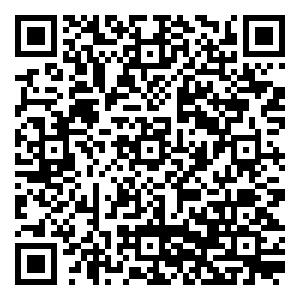
计量
- 文章访问数: 49
- HTML全文浏览量: 21
- 被引次数: 0