Multi-target Robust Prediction Model for Furnace Temperature and Flue Gas Oxygen Content in Municipal Solid Waste Incineration Process
-
摘要: 为实现城市固废焚烧过程炉温与烟气含氧量的准确预测, 提出一种基于改进随机配置网络($ \text{SCN} $)的多目标鲁棒建模方法. 首先, 设计了一种并行方式增量构建 SCN 隐含层, 通过信息叠加与跨越连接来增强隐含层映射多样性, 并利用参数自适应变化的监督不等式分配隐含层参数; 其次, 使用$ \text{F} $范数与$ L_{2,1} $范数正则项建立矩阵弹性网对模型参数进行稀疏约束, 以建模炉温与烟气含氧量间的相关性; 接着, 采用混合拉普拉斯分布作为每个目标建模误差的先验分布, 通过最大后验估计重新评估 SCN 模型的输出权值, 以增强其鲁棒性; 最后, 利用城市固废焚烧过程的历史数据对所提建模方法的性能进行测试. 实验结果表明, 所提建模方法在预测精度与鲁棒性方面具有优势.Abstract: To achieve accurate prediction of furnace temperature and flue gas oxygen content in municipal solid waste incineration process, a multi-target robust modeling method based on improved stochastic configuration network (SCN) is proposed. First, a parallel method is designed to incrementally build SCN hidden layers, which enhances the diversity of hidden layer mapping through information superposition and spanning connection, and assign hidden layer parameters using the supervised inequality with adaptive parameter changes. Second, a matrix elastic net is established by using F-norm and $ L_{2,1} $-norm regularization terms to sparsely constrain the model parameters to model the correlation between furnace temperature and flue gas oxygen content. Then, the mixture Laplace distribution is used as the prior distribution of each target modeling error, and the output weights of the SCN model are re-evaluated by maximum a posteriori estimation to enhance its robustness. Finally, the performance of the proposed modeling method is tested on the historical data of municipal solid waste incineration process. The experimental results show that the proposed modeling method has advantages in prediction accuracy and robustness.
-
表 1 MRI-SCN与不同类型建模方法在原始数据集上的对比实验结果
Table 1 Results of experiments comparing MRI-SCN with the different type of modeling methods on the original dataset
数据集 BP RBF RVFL MLS-SVR MRI-SCN 春季 5.80; 4.57; 86.57 5.40; 3.74; 89.14 4.55; 3.59; 92.36 3.41; 2.38; 95.70 3.12; 2.34; 96.38 夏季 5.63; 4.33; 87.48 4.85; 3.75; 91.49 4.60; 3.63; 92.14 3.37; 2.52; 95.90 3.16; 2.26; 96.25 秋季 5.39; 4.27; 89.03 4.92; 3.73; 91.25 4.52; 3.55; 92.44 3.44; 2.69; 95.41 3.08; 2.31; 96.48 冬季 5.30; 4.19; 89.93 5.72; 4.42; 89.14 4.96; 3.91; 91.46 3.52; 2.53; 95.61 3.10; 2.33; 96.68 表 2 MRI-SCN 与同类型建模方法在原始数据集上的对比实验结果
Table 2 Results of experiments comparing MRI-SCN with the same type of modeling methods on the original dataset
数据集 SCN MI-SCN MT-SCN MoGL-SCN MRI-SCN 春季 3.67; 2.86; 95.07 3.45; 2.64; 95.62 3.46; 2.67; 95.59 3.61; 2.68; 94.50 3.12; 2.34; 96.38 夏季 3.70; 2.80; 95.40 3.43; 2.55; 95.65 3.32; 2.57; 95.84 3.40; 2.67; 95.63 3.16; 2.26; 96.25 秋季 3.63; 2.73; 95.17 3.31; 2.56; 95.91 3.34; 2.61; 95.92 3.52; 2.76; 95.42 3.08; 2.31; 96.48 冬季 3.74; 2.94; 95.23 3.56; 2.73; 95.33 3.49; 2.81; 95.84 3.55; 2.31; 95.82 3.10; 2.33; 96.68 表 3 四组噪声数据集上的实验结果
Table 3 Results of experiments on the four noisy datasets
数据集 $ \zeta $ SCN MI-SCN MT-SCN MoGL-SCN MRI-SCN 10% 6.62; 5.15; 83.95 5.80; 4.57; 87.67 5.59; 4.40; 88.55 3.86; 2.87; 94.43 3.65; 2.74; 95.07 15% 7.59; 5.97; 78.84 6.39; 5.07; 84.97 6.35; 5.05; 85.16 4.00; 2.95; 94.02 3.87; 2.89; 94.45 春季 20% 8.96; 7.11; 70.38 7.33; 5.80; 80.14 7.36; 5.88; 79.99 4.26; 3.11; 93.21 4.03; 3.02; 93.95 25% 10.38; 8.05; 60.18 8.26; 6.46; 74.83 8.58; 6.74; 72.80 4.48; 3.26; 92.53 4.34; 3.24; 92.95 30% 11.17; 8.71; 53.98 8.70; 6.86; 72.21 9.40; 7.41; 67.46 4.82; 3.50; 91.25 4.56; 3.38; 92.28 10% 6.31; 4.90; 85.43 5.77; 4.56; 87.74 5.50; 4.30; 88.88 4.00; 2.93; 93.81 3.76; 2.78; 94.66 15% 7.38; 5.71; 79.98 6.48; 5.13; 84.56 6.24; 4.89, 85.75 4.38; 3.14; 92.66 3.96; 2.92; 94.07 夏季 20% 8.91; 7.00; 70.13 7.45; 5.94; 79.13 7.49; 5.96; 78.73 4.38; 3.17; 92.55 4.22; 3.13; 93.10 25% 9.40; 7.48; 66.62 7.89; 6.34; 76.58 7.93; 6.35; 76.22 4.68; 3.33; 91.83 4.45; 3.27; 92.43 30% 10.39; 8.21; 59.58 8.61; 6.90; 72.39 8.85; 7.09; 70.72 5.08; 3.65; 89.94 4.65; 3.41; 91.83 10% 6.40; 5.04; 85.18 5.98; 4.79; 86.83 5.57; 4.43; 88.69 3.73; 2.75; 94.76 3.46; 2.60; 95.53 15% 7.33; 5.72; 80.40 6.33; 5.01; 85.27 6.10; 4.78; 86.37 3.80; 2.80; 94.63 3.61; 2.72; 95.11 秋季 20% 8.90; 6.85; 71.15 7.19; 5.66; 80.85 7.40; 5.74; 79.96 4.03; 2.95; 94.02 3.86; 2.88; 94.45 25% 9.82; 7.52; 65.08 7.62; 6.00; 78.77 8.00; 6.20; 76.81 4.11; 2.98; 93.71 3.96; 2.96; 94.16 30% 10.79; 8.25; 57.89 8.28; 6.44; 74.97 8.75; 6.73; 72.24 4.50; 3.22; 92.52 4.26; 3.18; 93.28 10% 6.86; 5.34; 83.29 6.55; 5.16; 84.72 6.22; 4.86; 86.29 4.10; 3.04; 94.20 3.93; 2.98; 94.56 15% 7.94; 6.20; 78.14 7.27; 5.76; 81.77 6.97; 5.50; 83.25 4.40; 3.21; 93.30 4.27; 3.18; 93.73 冬季 20% 9.40; 7.36; 69.16 8.05; 6.32; 77.44 7.91; 6.20; 78.23 4.55; 3.33; 92.83 4.37; 3.28; 93.32 25% 10.56; 8.18; 60.47 8.81; 6.94; 72.48 8.90; 6.97; 71.96 4.88; 3.56; 91.40 4.62; 3.45; 92.41 30% 11.26; 8.74; 55.94 9.50; 7.48; 68.70 9.65; 7.57; 67.57 5.02; 3.64; 91.25 4.83; 3.60; 91.72 表 4 不同建模方法运行 30 次的时间对比
Table 4 Comparison of time for 30 runs of different modeling methods
方法 BP RBF SCN MI-SCN MT-SCN MoGL-SCN MRI-SCN 时间(s) 21.43 31.70 5.23 16.29 36.22 37.78 28.17 A1 多目标鲁棒预测模型输入变量明细
A1 Input variable details of multi-target robust prediction model
序号 变量名称 单位 1 进料器左内侧速度 % 2 进料器左外侧速度 % 3 进料器右内侧速度 % 4 进料器右外侧速度 % 5 干燥炉排左内侧速度 % 6 干燥炉排左外侧速度 % 7 干燥炉排右内侧速度 % 8 干燥炉排右外侧速度 % 9 干燥炉排左1空气流量 $ {\rm {km^3N/h}} $ 10 干燥炉排右1空气流量 $ {\rm {km^3N/h}} $ 11 干燥炉排左2空气流量 $ {\rm {km^3N/h}} $ 12 干燥炉排右2空气流量 $ {\rm {km^3N/h}} $ 13 燃烧炉排左1-1段空气流量 $ {\rm {km^3N/h}} $ 14 燃烧炉排右1-1段空气流量 $ {\rm {km^3N/h}} $ 15 燃烧炉排左1-2段空气流量 $ {\rm {km^3N/h}} $ 16 燃烧炉排右1-2段空气流量 $ {\rm {km^3N/h}} $ 17 燃烧炉排左2-1段空气流量 $ {\rm {km^3N/h}} $ 18 燃烧炉排右2-1段空气流量 $ {\rm {km^3N/h}} $ 19 燃烧炉排左2-2段空气流量 $ {\rm {km^3N/h}} $ 20 燃烧炉排右2-2段空气流量 $ {\rm {km^3N/h}} $ 21 燃烬炉排左空气流量 $ {\rm {km^3N/h}} $ 22 燃烬炉排右空气流量 $ {\rm {km^3N/h}} $ 23 二次风量 $ {\rm {km^3N/h}} $ 24 一次风机出口空气压力 kPa 25 一次空气加热器出口空气温度 ℃ 26 干燥炉排左内侧温度 ℃ 27 干燥炉排左外侧温度 ℃ 28 干燥炉排右内侧温度 ℃ 29 干燥炉排右外侧温度 ℃ 30 燃烧炉排1-1段左内侧温度 ℃ 31 燃烧炉排1-1段左外侧温度 ℃ 32 燃烧炉排1-1段右内侧温度 ℃ 33 燃烧炉排1-1段右外侧温度 ℃ 34 燃烧炉排1-2段左内侧温度 ℃ 35 燃烧炉排1-2段左外侧温度 ℃ 36 燃烧炉排1-2段右内侧温度 ℃ 37 燃烧炉排1-2段右外侧温度 ℃ 38 燃烧炉排2-1段左内侧温度 ℃ 39 燃烧炉排2-1段左外侧温度 ℃ 40 燃烧炉排2-1段右内侧温度 ℃ 41 燃烧炉排2-1段右外侧温度 ℃ 42 燃烧炉排2-2段左内侧温度 ℃ 43 燃烧炉排2-2段左外侧温度 ℃ 44 燃烧炉排2-2段右内侧温度 ℃ 45 燃烧炉排2-2段右外侧温度 ℃ 46 当前时刻的炉温 ℃ 47 当前时刻的烟气含氧量 % -
[1] Li Y, Zhao X G, Li Y B, Li X. Waste incineration industry and development policies in China. Waste Management, 2015, 46(8): 234−241 [2] 汤健, 夏恒, 余文, 乔俊飞. 城市固废焚烧过程智能优化控制研究现状与展望. 自动化学报, 2023, 49(10): 2019−2059Tang Jian, Xia Heng, Yu Wen, Qiao Jun-Fei. Research status and prospects of intelligent optimization control for municipal solid waste incineration process. Acta Automatica Sinica, 2023, 49(10): 2019−2059 [3] 严爱军, 胡开成. 城市生活垃圾焚烧炉温控制的多目标优化设定方法. 控制理论与应用, 2023, 40(4): 693−701Yan Ai-Jun, Hu Kai-Cheng. Multi-objective optimization setting method for temperature control of municipal solid waste incinerator. Control Theory & Applications, 2023, 40(4): 693−701 [4] Sun R, Ismail T M, Ren X H, Abd El-Salam M. Numerical and experimental studies on effects of moisture content on combustion characteristics of simulated municipal solid wastes in a fixed bed. Waste Management, 2015, 39(5): 166−178 [5] Magnanelli E, Tranås O L, Carlsson P, Mosby J, Becidan M. Dynamic modeling of municipal solid waste incineration. Energy, 2020, 299(10): 118426 [6] 蒋珂, 蒋朝辉, 谢永芳, 潘冬, 桂卫华. 基于动态注意力深度迁移网络的高炉铁水硅含量在线预测方法. 自动化学报, 2023, 49(5): 949−963Jiang Ke, Jiang Zhao-Hui, Xie Yong-Fang, Pan Dong, Gui Wei-Hua. Online prediction method for silic on content of molten iron in blast furnace based on dynamic attention deep transfer network. Acta Automatica Sinica, 2023, 49(5): 949−963 [7] Zhou X F, Zhai N J, Li S A, Shi H B. Time series prediction method of industrial process with limited data based on transfer learning. IEEE Transactions on Industrial Informatics, 2023, 19(5): 6872−6882 doi: 10.1109/TII.2022.3191980 [8] He H J, Meng X, Tang J, Qiao J F. A novel self-organizing T S fuzzy neural network for furnace temperature prediction in MSWI process. Neural Computing and Applications, 2022, 34(12): 9759−9776 [9] 郭海涛, 汤健, 丁海旭, 乔俊飞. 基于混合数据增强的MSWI 过程燃烧状态识别. 自动化学报, DOI: 10.16383/j.aas.c210843Guo Hai-Tao, Tang Jian, Ding Hai-Xu, Qiao Jun-Fei. Combustion states recognition method of mswi process based on mixed data enhancement. Acta Automatica Sinica, DOI: 10.16383/j.aas.c210843 [10] Qiao J F, Sun J, Meng X. Event-triggered adaptive model predictive control of oxygen content for municipal solid waste incineration process. IEEE Transactions on Automation Science and Engineering, 2024, 21(1): 463−474 doi: 10.1109/TASE.2022.3227918 [11] Pao Y H, Park G H, Sobajic D J. Learning and generalization characteristics of random vector functional-link net. Neurocomputing, 1994, 6(2): 163−180 doi: 10.1016/0925-2312(94)90053-1 [12] Wang D H, Li M. Stochastic configuration networks: Fundamentals and algorithms. IEEE Transactions on Cybernetics, 2017, 47(10): 3346−3479 [13] Li K, Yang C C, Wang W, Qiao J F. An improved stochastic configuration network for concentration prediction in wastewater treatment process. Information Sciences, 2023, 622(4): 148−160 [14] Li X, He Y, Ding J, Luan F, Zhang D. Predicting hot-strip finish rolling thickness using stochastic configuration networks. Information Sciences, 2022, 611(9): 677−689 [15] Lu J, Ding J L. Construction of prediction intervals for carbon residual of crude oil based on deep stochastic configuration networks. Information Sciences, 2019, 486(6): 119−132 [16] 胡开成, 严爱军, 王殿辉. 城市固废焚烧过程炉温非线性模型预测控制 [Online], available: http://kns.cnki.net/kcms/detail/44.1240.TP.20230330.0900.006.html, 2024-03-22Hu Kai-Cheng, Yan Ai-Jun, Wang Dian-Hui. Nonlinear model predictive control of furnace temperature for a municipal solid waste incineration process [Online], available: http://kns.cnki.net/kcms/detail/44.1240.TP.20230330.0900.006.html, March 22, 2024 [17] Yan A J, Guo J C, Wang D H. Heterogeneous feature ensemble modeling with stochastic configuration networks for predicting furnace temperature of a municipal solid waste incineration process. Neural Computing and Applications, 2022, 34(18): 15807−15819 doi: 10.1007/s00521-022-07271-9 [18] Ding H X, Tang J, Qiao J F. MIMO modeling and multi-loop control based on neural network for municipal solid waste incineration. Control Engineering Practice, 2022, 127(10): 105280 [19] Borchani H, Varando G, Bielza C, Larrañaga P. A survey on multi-output regression. Wiley Interdisciplinary Reviews Data Mining & Knowledge Discovery, 2015, 5(5): 216−233 [20] Kiliçarslan S, Közkurt C, Baş S, Elen A. Detection and classification of pneumonia using novel superior exponential (SupEx) activation function in convolutional neural networks. Expert Systems with Applications, 2023, 217(5): 119503 [21] Gao Z, Yu W, Yan J. Neuro adaptive fault-tolerant control embedded with diversified activating functions with application to auto-driving vehicles under fading actuation. IEEE Transactions on Neural Networks and Learning Systems, DOI: 10.1109/TNNLS.2023.3248100 [22] Li F J, Qiao J F, Han H G, Yang C L. A self-organizing cascade neural network with random weights for nonlinear system modeling. Applied Soft Computing, 2016, 42(5): 184−193 [23] Luo H Y, Han G L, Wu X T, Liu P X, Yang H, Zhang X. Cascaded hourglass feature fusing network for saliency detection. Neurocomputing, 2021, 428(3): 206−217 [24] Li J P, Hua C C, Qian J L, Guan X P. Low-rank based multi-input multi-output Takagi-Sugeno fuzzy modeling for prediction of molten iron quality in blast furnace. Fuzzy Sets and System, 2021, 421(9): 178−192 [25] Arashloo S R, Kittler J. Multi-target regression via non-linear output structure learning. Neurocomputing, 2022, 492(7): 572−580 [26] Tak N, İnan D. Type-1 fuzzy forecasting functions with elastic net regularization. Expert Systems with Applications, 2022, 199(8): 116916 [27] Nie F P, Huang H, Cai X, Ding C. Efficient and robust feature selection via joint L2, 1-norms minimization. In: Proceedings of Advances in Neural Information Processing Systems. Vancouver, Canada: MIT Press, 2010. 1813−1821 [28] Xiang S M, Nie F P, Meng G F, Pan C H, Zhang C S. Discriminative least squares regression for multiclass classification and feature selection. IEEE Transactions on Neural Networks and Learning Systems, 2012, 23(11): 1738−1754 doi: 10.1109/TNNLS.2012.2212721 [29] Li Y H, Hu L, Gao W F. Multi-label feature selection via robust flexible sparse regularization. Pattern Recognition, 2023, 134(2): 109074 [30] Lv S H, Zhao H Q, Zhou L J. Robust proportionate normalized least mean M-estimate algorithm for block-sparse system identification. IEEE Transactions on Circuits and Systems-II Express Briefs, 2022, 69(1): 234−238 doi: 10.1109/TCSII.2021.3082425 [31] Wang Q, He X, Jiang X, Li X L. Robust bi-stochastic graph regularized matrix factorization for data clustering. IEEE Transactions on Pattern Analysis and Machine Intelligence, 2022, 44(1): 390−403 [32] Yang X Y, Mu Y F, Cao K, Lv M Z, Peng B, Zhang Y, Wang G. Robust kernel recursive adaptive filtering algorithms based on M-estimate. Signal Processing, 2023, 207(6): 108952 [33] Duong N C, Speyer J L, Idan M. Laplace estimation for scalar linear systems. Automatica, 2022, 144(10): 110301 [34] Liang Z Z, Zhang L. L1-norm discriminant analysis via Bhattacharyya error bounds under Laplace distributions. Pattern Recognition, 2023, 141(9): 109609 [35] Lu J, Ding J L. Mixed-distribution based robust stochastic configuration networks for prediction interval construction. IEEE Transactions on Industrial Informatics, 2020, 16(8): 5099−5019 doi: 10.1109/TII.2019.2954351 [36] Song W X, Yao W X, Xing Y R. Robust mixture regression model fitting by Laplace distribution. Computational Statistics and Data Analysis, 2014, 71(3): 128−137 [37] Phillips R F. Least absolute deviations estimation via the EM algorithm. Statistics and Computing, 2002, 12(3): 281−285 doi: 10.1023/A:1020759012226 [38] Xu S, An X, Qiao X D, Zhu L J, Li L. Multi-output least-squares support vector regression machines. Pattern Recognition Letters, 2013, 34(9): 1078−1084 doi: 10.1016/j.patrec.2013.01.015 [39] Wang Q J, Hong Q Q, Wu S, Dai W. Multi-target stochastic configuration network and applications. IEEE Transactions on Artificial Intelligence, 2023, 4(2): 338−348 doi: 10.1109/TAI.2022.3162570 -
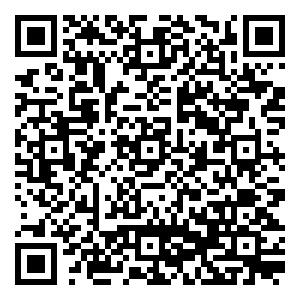
计量
- 文章访问数: 61
- HTML全文浏览量: 24
- 被引次数: 0