-
摘要: 事件抽取是一个历史悠久且极具挑战的研究任务, 取得了大量优异的成果. 由于事件抽取涉及的研究内容较多, 它们的目标和重心各不相同, 使得读者难以全面地了解事件抽取包含的研究任务、研究问题以及未来的热点趋势. 尽管现有的少量事件抽取综述梳理了相关成果, 但存在以下局限: 1)研究任务及其研究进展的梳理不清晰; 2)仅从技术路线的角度进行梳理. 由于不同研究任务下的不同研究问题的解决技术不宜一起对比, 因此这样的梳理方式不利于清晰地展示事件抽取在不同方面的研究进展情况. 为此, 面向研究问题对基于深度学习的事件抽取研究成果重新回顾整理. 首先, 界定事件的相关概念, 论述事件抽取的研究任务, 明确各研究任务的目标, 再梳理各任务上的代表性研究成果; 然后, 总结现有事件抽取成果主要致力于解决哪些方面的研究问题, 分析为什么会存在这些问题、为什么需要解决这些问题的原因; 紧接着对每个方面的研究问题进行技术路线梳理, 分析各自的大体研究方案以及研究推进的过程. 最后, 讨论事件抽取可能的发展趋势.Abstract: Event extraction is a long-standing and challenging task in natural language processing and has achieved encouraging results. Given various research targets and concerns, it is difficult for readers to comprehensively understand the situations and trends of event extraction. Although limited survey has reviewed related results on event extraction, it shares two limitations, including 1) insufficient depiction for research tasks and development, and 2) focusing on technical classification instead of research issues. Since it is not suitable to compare the techniques of various research issues under different research tasks, such a combing method is not conducive to clearly demonstrate the research development of diverse aspects of event extraction. To tackle these weaknesses, we review event extraction studies from the perspectives of research tasks, research issues and corresponding solving methods. Specifically, the event definition is discussed first, followed by an elaborate description and analysis for research tasks to clarify the targets of diverse research tasks. Meanwhile, we hackle the representative research results. Then, we summarize what aspects of event extraction the existing studies are mainly devoted to solving, and analyze why these issues exist and why they need to be addressed. Subsequently, the technical line of each aspect is sorted out to investigate the development and advancement of each other. Finally, we explore the future direction of event extraction.
-
Key words:
- Event extraction /
- research tasks /
- research development and solutions /
- deep learning
-
表 1 各模型在ChFinAnn语料上各事件类型下的F1值 (%)
Table 1 F1 scores of models under each event type on ChFinAnn corpus (%)
模型 冻结 回购 减持 增持 质押 平均 DCFEE-O 51.1 83.1 45.3 46.6 63.9 58.0 DCFEE-M 45.6 80.8 44.2 44.9 62.9 55.7 Greedy-Dec 58.9 78.9 51.2 51.3 62.1 60.5 Doc2EDAG 70.2 87.3 71.8 75.0 77.3 76.3 GIT 73.4 90.8 74.3 76.3 77.7 78.5 DE-PPN 73.5 87.4 74.4 75.8 78.4 77.9 SCDEE 80.4 90.5 75.1 70.1 78.1 78.8 PTPCG 71.4 91.6 71.5 72.2 76.4 76.6 ReDEE 74.1 90.7 75.3 78.1 80.1 79.7 TER-MCEE 87.9 97.2 89.8 91.2 78.6 88.9 EDEE 97.4 90.3 93.2 93.4 96.2 94.1 ProCNet 75.7 93.7 76.0 72.0 81.3 79.7 表 2 处理训练语料不足问题的各方法对比
Table 2 Comparison of methods that handling the problem of insufficient training corpus
方法 本质 需要的数据 解决方式 远程监督 利用外部知识库扩展数据 少量标注数据 直接增加 半监督 少量标注训练模型预测大量无标签数据 少量标注数据+大量无标签数据 直接增加、不增加 无监督 直接根据数据特点或性质判断 大量无标签数据 无标注数据 自监督 从无标签数据中挖掘监督信息用于训练 大量无标签数据 无标注数据 弱监督 针对数据集不可靠情况, 包含3种典型情况 少量标注数据+大量无标签数据 直接增加 主动学习 通过机器学习挑选有用的样本给人工标注 少量标注数据+大量无标签数据 直接增加 强化学习 中途告知学习情况 大量无标签数据 无标注数据 元学习 通过多个任务的数据学习内涵/规律/学习的本领 其他任务或领域的数据 其他领域增加 迁移学习 其它任务/领域下的模型用于目标任务/领域 其它领域的大量数据 其他领域增加 少样本学习 一种任务, 少样本下学习本领 极少的标注数据 直接增加、间接增加、不增加、其他领域数据 零样本学习 一种任务, 零样本下学习本领 给出代表某一类物体语义的嵌入向量 无标注数据 -
[1] Liu K, Chen Y B, Liu J, Zuo X Y, Zhao J. Extracting events and their relations from texts: A survey on recent research progress and challenges. AI Open, 2020, 1: 22–39 doi: 10.1016/j.aiopen.2021.02.004 [2] 王县县, 禹龙, 田生伟, 王瑞锦. 独立RNN 和胶囊网络的维吾尔语事件缺失元素填充. 自动化学报, 2021, 47(4): 903–912Wang Xian-Xian, Yu Long, Tian Sheng-Wei, Wang Rui-Jin. Missing argument fllling of uyghur event based on independent recurrent neural network and capsule network. Acta Automatica Sinica, 2021, 47(4): 903–912 [3] 王梦来, 李想, 陈奇, 李澜博, 赵衍运. 基于CNN的监控视频事件检测. 自动化学报, 2016, 42(6): 892–903 doi: 10.16383/j.aas.2016.c150729Wang Meng-Lai, Li Xiang, Chen Qi, Li Lan-Bo, Zhao Yan-Yun. Surveillance event detection based on CNN. Acta Automatica Sinica, 2016, 42(6): 892–903 doi: 10.16383/j.aas.2016.c150729 [4] 介飞, 谢飞, 李磊, 吴信东. 社交网络中隐式事件突发性检测. 自动化学报, 2018, 44(4): 730–742 doi: 10.16383/j.aas.2017.c160564Jie Fei, Xie Fei, Li Lei, Wu Xin-Dong. Latent event-related burst detection in social networks. Acta Automatica Sinica, 2018, 44(4): 730–742 doi: 10.16383/j.aas.2017.c160564 [5] Valenzuela-Escarcega M A, Hahn-Powell G, Surdeanu M, Hicks T. A domain-independent rule-based framework for event extraction. In: Proceedings of the 53rd Annual Meeting of the Association for Computational Linguistics and the 7th International Joint Conference on Natural Language Processing of the Asian Federation of Natural Language Processing. Beijing, China: Association for Computational Linguistics, 2015. 127–132 [6] Sha L, Liu J, Lin C Y, Li S J, Chang B B, Sui Z F. RBPB: Regularization-based pattern balancing method for event extraction. In: Proceedings of the 54th Annual Meeting of the Association for Computational Linguistics. Berlin, Germany: Association for Computational Linguistics, 2016. 1224–1234 [7] Hsi A, Yang Y M, Carbonell J, Xu R C. Leveraging multilingual training for limited resource event extraction. In: Proceedings of the 26th International Conference on Computational Linguistics: Technical Papers. Osaka, Japan: Association for Computational Linguistics, 2016. 1201–1210 [8] Li F, Zhang Y, Zhang M S, Ji D H. Joint models for extracting adverse drug events from biomedical text. In: Proceedings of the 25th International Joint Conference on Artificial Intelligence. New York, USA: Springer, 2016. 2838–2844 [9] Nguyen T H, Cho K, Grishman R. Joint event extraction via recurrent neural networks. In: Proceedings of the Conference of the North American Chapter of the Association for Computational Linguistics: Human Language Technologies. San Diego, USA: Association for Computational Linguistics, 2016. 300–309 [10] Badgett A, Huang R H. Extracting subevents via an effective two-phase approach. In: Proceedings of the Conference on Empirical Methods in Natural Language Processing. Austin, USA: Association for Computational Linguistics, 2016. 906–911 [11] Chen Y B, Liu S L, Zhang X, Liu K, Zhao J. Automatically labeled data generation for large scale event extraction. In: Proceedings of the 55th Annual Meeting of the Association for Computational Linguistics. Vancouver, Canada: Association for Computational Linguistics, 2017. 409–419 [12] Sha L, Qian F, Chang B B, Sui Z F. Jointly extracting event triggers and arguments by dependency-bridge RNN and tensor-based argument interaction. In: Proceedings of the 32nd AAAI Conference on Artificial Intelligence. New Orleans, USA: AAAI, 2018. 5916–5923 [13] Zeng Y, Feng Y S, Ma R, Wang Z, Yan R, Shi C D, et al. Scale up event extraction learning via automatic training data generation. In: Proceedings of the 32nd AAAI Conference on Artificial Intelligence. New Orleans, USA: AAAI, 2018. 6045–6052 [14] Huang L F, Ji H, Cho K, Dagan I, Riedel S, Voss C R. Zero-shot transfer learning for event extraction. In: Proceedings of the 56th Annual Meeting of the Association for Computational Linguistics. Melbourne, Australia: Association for Computational Linguistics, 2018. 2160–2170 [15] Liu X, Luo Z C, Huang H Y. Jointly multiple events extraction via attention-based graph information aggregation. In: Proceedings of the Conference on Empirical Methods in Natural Language Processing. Brussels, Belgium: Association for Computational Linguistics, 2018. 1247–1256 [16] Subburathinam A, Lu D, Ji H, May J, Chang S F, Sil A, et al. Cross-lingual structure transfer for relation and event extraction. In: Proceedings of the Conference on Empirical Methods in Natural Language Processing and the 9th International Joint Conference on Natural Language Processing. Hong Kong, China: Association for Computational Linguistics, 2019. 313–325 [17] Zhang J C, Qin Y X, Zhang Y, Liu M C, Ji D H. Extracting entities and events as a single task using a transition-based neural model. In: Proceedings of the 28th International Joint Conference on Artificial Intelligence. Macao, China: Springer, 2019. 5422–5428 [18] Yang S, Feng D W, Qiao L B, Kan Z G, Li D S. Exploring pre-trained language models for event extraction and generation. In: Proceedings of the 57th Annual Meeting of the Association for Computational Linguistics. Florence, Italy: Association for Computational Linguistics, 2019. 5284–5294 [19] 贺瑞芳, 段邵杨. 基于多任务学习的中文事件抽取联合模型. 软件学报, 2019, 30(4): 1015–1030 doi: 10.13328/j.cnki.jos.005380He Rui-Fang, Duan Shao-Yang. Joint Chinese event extraction based multi-task learning. Journal of Software, 2019, 30(4): 1015–1030 doi: 10.13328/j.cnki.jos.005380 [20] Li D Y, Huang L F, Ji H, Han J W. Biomedical event extraction based on knowledge-driven tree-LSTM. In: Proceedings of the Conference of the North American Chapter of the Association for Computational Linguistics: Human Language Technologies. Minneapolis, USA: Association for Computational Linguistics, 2019. 1421–1430 [21] Huang P X, Zhao X, Takanobu R, Zhen T, Xiao W D. Joint event extraction with hierarchical policy network. In: Proceedings of the 28th International Conference on Computational Linguistics. Barcelona, Spain: Association for Computational Linguistics, 2020. 2653–2664 [22] Du X Y, Cardie C. Event extraction by answering (almost) natural questions. In: Proceedings of the Conference on Empirical Methods in Natural Language Processing. Virtual Event: Association for Computational Linguistics, 2020. 671–683 [23] Li F Y, Peng W H, Chen Y G, Wang Q, Pan L, Lyu Y J, et al. Event extraction as multi-turn question answering. In: Proceedings of the Conference on Empirical Methods in Natural Language Processing. Virtual Event: Association for Computational Linguistics, 2020. 829–838 [24] Liu J, Chen Y B, Liu K, Bi W, Liu X J. Event extraction as machine reading comprehension. In: Proceedings of the Conference on Empirical Methods in Natural Language Processing. Virtual Event: Association for Computational Linguistics, 2020. 1641–1651 [25] Ma J, Wang S, Anubhat R, Ballesteros M, Al-Onaizan Y. Resource-enhanced neural model for event argument extraction. In: Findings of the Association for Computational Linguistics: EMNLP. Virtual Event: Association for Computational Linguistics, 2020. 3554–3559 [26] Abdulkadhar S, Bhasuran B, Natarajan J. Multiscale Laplacian graph kernel combined with lexico-syntactic patterns for biomedical event extraction from literature. Knowledge and Information Systems, 2021, 63: 143–173 doi: 10.1007/s10115-020-01514-8 [27] Li Q, Peng H, Li J X, Wu J, Ning Y X, Wang L H, et al. Reinforcement learning-based dialogue guided event extraction to exploit argument relations. IEEE/ACM Transactions on Audio, Speech, and Language Processing, 2022, 30: 520–533 doi: 10.1109/TASLP.2021.3138670 [28] Zheng S, Cao W, Xu W, Bian J. Doc2EDAG: An end-to-end document-level framework for Chinese financial event extraction. In: Proceedings of the Conference on Empirical Methods in Natural Language Processing and the 9th International Joint Conference on Natural Language Processing. Hong Kong, China: Association for Computational Linguistics, 2019. 337–346 [29] Li S, Ji H, Han J W. Document-level event argument extraction by conditional generation. In: Proceedings of the Conference of the North American Chapter of the Association for Computational Linguistics: Human Language Technologies. Virtual Event: Association for Computational Linguistics, 2021. 894–908 [30] Du X Y, Cardie C. Document-level event role filler extraction using multi-granularity contextualized encoding. In: Proceedings of the 58th Annual Meeting of the Association for Computational Linguistics. Virtual Event: Association for Computational Linguistics, 2020. 8010–8020 [31] Zhao Y, Jin X L, Wang Y Z, Cheng X Q. Document embedding enhanced event detection with hierarchical and supervised attention. In: Proceedings of the 56th Annual Meeting of the Association for Computational Linguistics. Melbourne, Australia: Association for Computational Linguistics, 2018. 414–419 [32] Yang B S, Mitchell T. Joint extraction of events and entities within a document context. In: Proceedings of the Conference of the North American Chapter of the Association for Computational Linguistics: Human Language Technologies. San Diego, USA: Association for Computational Linguistics, 2016. 289–299 [33] Yang H, Chen Y B, Liu K, Xiao Y, Zhao J. DCFEE: A document-level Chinese financial event extraction system based on automatically labeled training data. In: Proceedings of the 56th Annual Meeting of the Association for Computational Linguistics-System Demonstrations. Melbourne, Australia: Association for Computational Linguistics, 2018. 1–6 [34] Zhao W Z, Zhang J Y, Yang J C, He T T, Ma H F, Li Z X. A novel joint biomedical event extraction framework via two-level modeling of documents. Information Sciences, 2021, 550: 27–40 doi: 10.1016/j.ins.2020.10.047 [35] Zhang Z S, Kong X, Liu Z Z, Ma X Z, Hovy E. A two-step approach for implicit event argument detection. In: Proceedings of the 58th Annual Meeting of the Association for Computational Linguistics. Virtual Event: Association for Computational Linguistics, 2020. 7479–7485 [36] Ebner S, Xia P, Culkin R, Rawlins K, Durme B V. Multi-sentence argument linking. In: Proceedings of the 58th Annual Meeting of the Association for Computational Linguistics. Virtual Event: Association for Computational Linguistics, 2020. 8057–8077 [37] Liu S L, Chen Y B, He S Z, Liu K, Zhao J. Leveraging framenet to improve automatic event detection. In: Proceedings of the 54th Annual Meeting of the Association for Computational Linguistics. Berlin, Germany: Association for Computational Linguistics, 2016. 2134–2143 [38] Zhang C, Soderland S, Weld D S. Exploiting parallel news streams for unsupervised event extraction. Transactions of the Association for Computational Linguistics, 2015, 3: 117–129 doi: 10.1162/tacl_a_00127 [39] Ferguson J, Lockard C, Weld D S, Hajishirzi H. Semi-supervised event extraction with paraphrase clusters. In: Proceedings of the Conference of the North American Chapter of the Association for Computational Linguistics: Human Language Technologies. New Orleans, USA: Association for Computational Linguistics, 2018. 359–364 [40] Liu J, Chen Y B, Liu K, Zhao J. Event detection via gated multilingual attention mechanism. In: Proceedings of the 32nd AAAI Conference on Artificial Intelligence. New Orleans, USA: AAAI, 2018. 4865–4872 [41] Lee K, Qadir A, Hasan S A, Datla V, PRAKASH A, LIU J, et al. Adverse drug event detection in tweets with semi-supervised convolutional neural networks. In: Proceedings of the Web Conference. Perth, Australia: ACM, 2017. 705–714 [42] Peng H R, Song Y Q, Roth D. Event detection and co-reference with minimal supervision. In: Proceedings of the Conference on Empirical Methods in Natural Language Processing. Austin, USA: Association for Computational Linguistics, 2016. 392–402 [43] Deng S M, Zhang N Y, Kang J J, Zhang Y C, Zhang W, Chen H J. Meta-learning with dynamic-memory-based prototypical network for few-shot event detection. In: Proceedings of the 13th International Conference on Web Search and Data Mining. Houston, USA: ACM, 2020. 151–159 [44] Lai V D, Nguyen M V, Nguyen T H, Dernoncourt F. Graph learning regularization and transfer learning for few-shot event detection. In: Proceedings of the 44th International ACM SIGIR Conference on Research and Development in Information Retrieval. Virtual Event: ACM, 2021. 2172–2176 [45] Yuan Q, Ren X, He W Q, Zhang C, Geng X H, Huang L F, et al. Open-schema event profiling for massive news corpora. In: Proceedings of the 27th ACM International Conference on Information and Knowledge Management. Torino, Italy: ACM, 2018. 587–596 [46] Shen S R, Qi G L, Li Z, Bi S, Wang L S. Hierarchical Chinese legal event extraction via pedal attention mechanism. In: Proceedings of the 28th International Conference on Computational Linguistics. Barcelona, Spain: Association for Computational Linguistics, 2020. 100–113 [47] Bhardwaj A, Yang J, Cudre-Mauroux P. A human-AI loop approach for joint keyword discovery and expectation estimation in micropost event detection. In: Proceedings of the 34th AAAI Conference on Artificial Intelligence. New York, USA: AAAI, 2020. 2451–2458 [48] Hogemnoom F, Frasincar F, Kaymak U, Jong F D, Caron E. A survey of event extraction methods from text for decision support systems. Decision Support Systems, 2016, 85: 12–22 doi: 10.1016/j.dss.2016.02.006 [49] Li Q, Li J X, Sheng J W, Cui S Y, Wu J, Hei Y M, et al. A survey on deep learning event extraction: Approaches and applications. IEEE Transactions on Neural Networks and Learning Systems, 2023, 34: 1–21 doi: 10.1109/TNNLS.2023.3305210 [50] 黄河燕, 刘啸. 面向新领域的事件抽取研究综述. 智能系统学报, 2022, 17(1): 201–212Huang He-Yan, Liu Xiao. A survey on event extraction in new domains. CAAI Transactions on Intelligent Systems, 2022, 17(1): 201–212 [51] Karisani P, Ho J C, Agichtein E. Domain-guided task decomposition with self-training for detecting personal events in social media. In: Proceedings of the Web Conference. Taipei, China: ACM, 2020. 2411–2420 [52] Yen A Z, Huang H H, Chen H H. Detecting personal life events from twitter by multi-task LSTM. In: Proceedings of the Web Conference. Lyon, France: ACM, 2018. 21–22 [53] Cao Y W, Peng H, Wu J, Dou Y T, Li J X, Yu P S. Knowledge-preserving incremental social event detection via heterogeneous GNNs. In: Proceedings of the Web Conference. Ljubljana, Slovenia: ACM, 2021. 3383–3395 [54] 万齐智, 万常选, 胡蓉, 刘德喜. 基于句法语义依存分析的中文金融事件抽取. 计算机学报, 2021, 44(3): 508–530 doi: 10.11897/SP.J.1016.2021.00508Wan Qi-Zhi, Wan Chang-Xuan, Hu Rong, Liu De-Xi. Chinese financial event extraction based on syntactic and semantic dependency parsing. Chinese Journal of Computers, 2021, 44(3): 508–530 doi: 10.11897/SP.J.1016.2021.00508 [55] Wan Q Z, Wan C X, Xiao K L, Hu R, Liu D X. A multi-channel hierarchical graph attention network for open event extraction. ACM Transactions on Information Systems, 2023, 41(1): 1–27 [56] Wakamiya S, Jatowt A, Kawat Y, Akiyama T. Analyzing global and pairwise collective spatial attention for geo-social event detection in microblogs. In: Proceedings of the Web Conference. Montreal, Canada: ACM, 2016. 263–266 [57] Satyapanich T, Ferraro F, Finin T. CASIE: Extracting cybersecurity event information from text. In: Proceedings of the 34th AAAI Conference on Artificial Intelligence. New York, USA: AAAI, 2020. 8749–8757 [58] Nguyen T H, Grishman R. Graph convolutional networks with argument-aware pooling for event detection. In: Proceedings of the 32nd AAAI Conference on Artificial Intelligence. New Orleans, USA: AAAI, 2018. 5900–5907 [59] Yan H R, Jin X L, Meng X B, Guo J F, Cheng X Q. Event detection with multi-order graph convolution and aggregated attention. In: Proceedings of the Conference on Empirical Methods in Natural Language Processing and the 9th International Joint Conference on Natural Language Processing. Hong Kong, China: Association for Computational Linguistics, 2019. 5766–5770 [60] Cui S Y, Yu B W, Liu T W, Zhang Z Y, Wang X B, Shi J Q. Edge-enhanced graph convolution networks for event detection with syntactic relation. In: Proceedings of the Conference on Empirical Methods in Natural Language Processing: Findings. Virtual Event: Association for Computational Linguistics, 2020. 2329–2339 [61] Lai V D, Nguyen T N, Nguyen T H. Event detection: Gate diversity and syntactic importance scores for graph convolution neural networks. In: Proceedings of the Conference on Empirical Methods in Natural Language Processing. Virtual Event: Association for Computational Linguistics, 2020. 5405–5411 [62] Veyseh A P B, Nguyen T N, Nguyen T H. Graph transformer networks with syntactic and semantic structures for event argument extraction. In: Proceedings of the Conference on Empirical Methods in Natural Language Processing: Findings. Virtual Event: Association for Computational Linguistics, 2020. 3651–3661 [63] Ahmad W U, Peng N Y, Chang K. GATE: Graph attention transformer encoder for cross-lingual relation and event extraction. In: Proceedings of the 35th AAAI Conference on Artificial Intelligence. Virtual Event: AAAI, 2021. 12462–12470 [64] Hong Y, Zhou W X, Zhang J L, Zhu Q M, Zhou G D. Self-regulation: Employing a generative adversarial network to improve event detection. In: Proceedings of the 56th Annual Meeting of the Association for Computational Linguistics. Melbourne, Australia: Association for Computational Linguistics, 2018. 515–526 [65] Wang X Z, Han X, Liu Z Y, Sun M S, Li P. Adversarial training for weakly supervised event detection. In: Proceedings of the Conference of the North American Chapter of the Association for Computational Linguistics: Human Language Technologies. Minneapolis, USA: Association for Computational Linguistics, 2019. 998–1008 [66] Huang K H, Hsu I H, Natarajan P, Chang K W, Peng N Y. Multilingual generative language models for zero-shot cross-lingual event argument extraction. In: Proceedings of the 60th Annual Meeting of the Association for Computational Linguistics. Dublin, Ireland: Association for Computational Linguistics, 2022. 4633–4646 [67] Arachie C, Gaur M, Aazaroot S, Groves W, Zhang K, Jaimes A. Unsupervised detection of sub-events in large scale disasters. In: Proceedings of the 34th AAAI Conference on Artificial Intelligence. New York, USA: AAAI, 2020. 354–361 [68] Xie J Y, Sun H T, Zhou J S, Qu W G, Dai X Y. Event detection as graph parsing. In: Findings of the Association for Computational Linguistics: ACL-IJCNLP. Virtual Event: Association for Computational Linguistics, 2021. 1630–1640 [69] Veyseh A P B, Lai V D, Dernoncourt F, Nguyen T H. Unleash GPT-2 power for event detection. In: Proceedings of the 59th Annual Meeting of the Association for Computational Linguistics and the 11th International Joint Conference on Natural Language Processing. Virtual Event: Association for Computational Linguistics, 2021. 6271–6282 [70] Liao J Z, Zhao X, Li X Y, Zhang L L, Tang J Y. Learning discriminative neural representations for event detection. In: Proceedings of the 44th International ACM SIGIR Conference on Research and Development in Information Retrieval. Virtual Event: ACM, 2021. 644–653 [71] Nguyen T H, Grishman R. Event detection and domain adaptation with convolutional neural networks. In: Proceedings of the 53rd Annual Meeting of the Association for Computational Linguistics and the 7th International Joint Conference on Natural Language Processing. Beijing, China: Association for Computational Linguistics, 2015. 365–371 [72] Chen Y B, Xu L H, Liu K, Zeng D J, Zhao J. Event extraction via dynamic multi-pooling convolutional neural networks. In: Proceedings of the 53rd Annual Meeting of the Association for Computational Linguistics and the 7th International Joint Conference on Natural Language Processing. Beijing, China: Association for Computational Linguistics, 2015. 167–176 [73] Nguyen T H, Grishman R. Modeling skip-grams for event detection with convolutional neural networks. In: Proceedings of the Conference on Empirical Methods in Natural Language Processing. Austin, USA: Association for Computational Linguistics, 2016. 886–891 [74] Ghaeini R, Fern X Z, Huang L, Tadepalli P. Event nugget detection with forward-backward recurrent neural networks. In: Proceedings of the 54th Annual Meeting of the Association for Computational Linguistics. Berlin, Germany: Association for Computational Linguistics, 2016. 369–373 [75] Liu S L, Chen Y B, Liu K, Zhao J. Exploiting argument information to improve event detection via supervised attention mechanisms. In: Proceedings of the 55th Annual Meeting of the Association for Computational Linguistics. Vancouver, Canada: Association for Computational Linguistics, 2017. 1789–1798 [76] Kipf T N, Welling M. Semi-supervised classification with graph convolutional networks. In: Proceedings of 5th International Conference on Learning Representations. Toulon, France: OpenReview.net, 2017. 1–14 [77] Liu S B, Cheng R, Yu X M, Cheng X Q. Exploiting contextual information via dynamic memory network for event detection. In: Proceedings of the Conference on Empirical Methods in Natural Language Processing. Brussels, Belgium: Association for Computational Linguistics, 2018. 1030–1035 [78] Mehta S, Islam M R, Rangwala H, Ramakrishnan N. Event detection using hierarchical multi-aspect attention. In: Proceedings of the Web Conference. San Francisco, USA: ACM, 2019. 3079–3084 [79] Xie Z P, Tu Y M. A graph convolutional network with adaptive graph generation and channel selection for event detection. In: Proceedings of the 36th AAAI Conference on Artificial Intelligence, Virtual Event: AAAI, 2022. 11522–11529 [80] Ghaeini R, Fern X Z, Huang L, Tadepalli P. Event nugget detection with forward-backward recurrent neural networks. In: Proceedings of the 54th Annual Meeting of the Association for Computational Linguistics. Berlin, Germany: Association for Computational Linguistics, 2016. 369–373 [81] Liu S L, Chen Y B, Liu K, Zhao J. Exploiting argument information to improve event detection via supervised attention mechanisms. In: Proceedings of the 55th Annual Meeting of the Association for Computational Linguistics. Vancouver, Canada: Association for Computational Linguistics, 2017. 1789–1798 [82] Kipf T N, Welling M. Semi-supervised classification with graph convolutional networks. In: Proceedings of 5th International Conference on Learning Representations. Toulon, France: OpenReview.net, 2017. 1–14 [83] Liu S B, Cheng R, Yu X M, Cheng X Q. Exploiting contextual information via dynamic memory network for event detection. In: Proceedings of the Conference on Empirical Methods in Natural Language Processing. Brussels, Belgium: Association for Computational Linguistics, 2018. 1030–1035 [84] Mehta S, Islam M R, Rangwala H, Ramakrishnan N. Event detection using hierarchical multi-aspect attention. In: Proceedings of the Web Conference. San Francisco, USA: ACM, 2019. 3079–3084 [85] Xie Z P, Tu Y M. A graph convolutional network with adaptive graph generation and channel selection for event detection. In: Proceedings of the 36th AAAI Conference on Artificial Intelligence, Virtual Event: AAAI, 2022. 11522–11529 [86] Liu J, Chen Y F, Xu J A. Saliency as evidence: Event detection with trigger saliency attribution. In: Proceedings of the 60th Annual Meeting of the Association for Computational Linguistics. Dublin, Ireland: Association for Computational Linguistics, 2022. 4573–4585 [87] Li H C, Mo T, Fan H C, Wang J K, Wang J X, Zhang F H, et al. KiPT: Knowledge-injected prompt tuning for event detection. In: Proceedings of the 29th International Conference on Computational Linguistics. Gyeongju, Korea: Association for Computational Linguistics, 2022. 1943–1952 [88] Yue Z R, Zeng H M, Lan M F, Ji H, Wang D. Zero- and few-shot event detection via prompt-based meta learning. In: Proceedings of the 61st Annual Meeting of the Association for Computational Linguistics. Toronto, Canada: Association for Computational Linguistics, 2023. 7928–7943 [89] Dai L, Wang B, Xiang W, Mo Y J. Bi-directional iterative prompt-tuning for event argument extraction. In: Proceedings of the Conference on Empirical Methods in Natural Language Processing. Abu Dhabi, United Arab Emirates: Association for Computational Linguistics, 2022. 6251–6263 [90] Nguyen C V, Man H, Nguyen T H. Contextualized soft prompts for extraction of event arguments. In: Findings of the Association for Computational Linguistics: ACL. Toronto, Canada: Association for Computational Linguistics, 2023. 4352–4361 [91] Wang S J, Yu M, Huang L F. The art of prompting: Event detection based on type specific prompts. In: Proceedings of the 61st Annual Meeting of the Association for Computational Linguistics. Toronto, Canada: Association for Computational Linguistics, 2023. 1286–1299 [92] Du X Y, Ji H. Retrieval-augmented generative question answering for event argument extraction. In: Proceedings of the Conference on Empirical Methods in Natural Language Processing. Abu Dhabi, United Arab Emirates: Association for Computational Linguistics, 2022. 4649–4666 [93] Hsu I H, Xie Z Y, Huang K H, Natarajan P, Peng N Y. AMPERE: AMR-aware prefix for generation-based event argument extraction model. In: Proceedings of the 61st Annual Meeting of the Association for Computational Linguistics. Toronto, Canada: Association for Computational Linguistics, 2023. 10976–10993 [94] Lu D, Ran S H, Tetreault J, Jaimes A. Event extraction as question generation and answering. In: Proceedings of the 61st Annual Meeting of the Association for Computational Linguistics. Toronto, Canada: Association for Computational Linguistics, 2023. 1666–1688 [95] Ma M D, Taylor A K, Wang W, Peng N Y. DICE: Data-efficient clinical event extraction with generative models. In: Proceedings of the 61st Annual Meeting of the Association for Computational Linguistics. Toronto, Canada: Association for Computational Linguistics, 2023. 15898–15917 [96] Bendimerad A, Plantevit M, Robardet C, Amer-Yahia S. User-driven geolocated event detection in social media. IEEE Transactions on Knowledge and Data Engineering, 2021, 33(2): 796–809 [97] Liu X W, Fu J M, Chen Y J. Event evolution model for cybersecurity event mining in tweet streams. Information Sciences, 2020, 524: 254–276 doi: 10.1016/j.ins.2020.03.048 [98] Choubey P K, Raju K, Huang R H. Identifying the most dominant event in a news article by mining event coreference relations. In: Proceedings of the Conference of the North American Chapter of the Association for Computational Linguistics: Human Language Technologies. New Orleans, USA: Association for Computational Linguistics, 2018. 340–345 [99] Qian Y, Deng X W, Ye Q W, Ma B J, Yuan H. On detecting business event from the headlines and leads of massive online news articles. Information Processing and Management, 2019, 56(6): Article No. 102086 doi: 10.1016/j.ipm.2019.102086 [100] Akbari M, Hu X, Nie L Q, Chua T S. From tweets to wellness: Wellness event detection from twitter streams. In: Proceedings of the 30th AAAI Conference on Artificial Intelligence. Phoenix, USA: AAAI, 2016. 87–93 [101] Ding H B, Riloff E. Acquiring knowledge of affective events from blogs using label propagation. In: Proceedings of the 30th AAAI Conference on Artificial Intelligence. Phoenix, USA: AAAI, 2016. 2935–2942 [102] Bendimerad A, Plantevit M, Robardet C, Amer-Yahia S. User-driven geolocated event detection in social media. IEEE Transactions on Knowledge and Data Engineering, 2021, 33(2): 796–809 [103] Liu X W, Fu J M, Chen Y J. Event evolution model for cybersecurity event mining in tweet streams. Information Sciences, 2020, 524: 254–276 [104] Choubey P K, Raju K, Huang R H. Identifying the most dominant event in a news article by mining event coreference relations. In: Proceedings of the Conference of the North American Chapter of the Association for Computational Linguistics: Human Language Technologies. New Orleans, USA: Association for Computational Linguistics, 2018. 340–345 [105] Qian Y, Deng X W, Ye Q W, Ma B J, Yuan H. On detecting business event from the headlines and leads of massive online news articles. Information Processing and Management, 2019, 56(6): Article No. 102086 [106] Chen Y B, Yang H, Liu K, Zhao J, Jia Y T. Collective event detection via a hierarchical and bias tagging networks with gated multi-level attention mechanisms. In: Proceedings of the Conference on Empirical Methods in Natural Language Processing. Brussels, Belgium: Association for Computational Linguistics, 2018. 1267–1276 [107] Veyseh A P B, Nguyen M V, Trung N N, Min B N, Nguyen H. Modeling document-level context for event detection via important context selection. In: Proceedings of the Conference on Empirical Methods in Natural Language Processing. Punta Cana, Dominican Republic: Association for Computational Linguistics, 2021. 5403–5413 [108] Veyseh A P, Nguyen M V, Dernoncourt F, Min B N, Nguyen T H. Document-level event argument extraction via optimal transport. In: Proceedings of the Findings of the Association for Computational Linguistics: ACL. Dublin, Ireland: Association for Computational Linguistics, 2022. 1648–1658 [109] Han C Y, Zhang J C, Li X Y, Xu G J, Peng W H, Zeng Z F. DuEE-Fin: A large-scale dataset for document-level event extraction. In: Proceedings of the CCF International Conference on Natural Language Processing and Chinese Computing. Guilin, China: Springer, 2022. 172–183 [110] Ma Y B, Wang Z H, Cao Y X, Li M K, Chen M Q, Wang K, et al. Prompt for extraction? PAIE: Prompting argument interaction for event argument extraction. In: Proceedings of the 60th Annual Meeting of the Association for Computational Linguistics. Dublin, Ireland: Association for Computational Linguistics, 2022. 6759–6774 [111] Lin J J, Chen Q, Zhou J, Jin J, He L. CUP: Curriculum learning based prompt tuning for implicit event argument extraction. In: Proceedings of the 31st International Joint Conference on Artificial Intelligence. Vienna, Austria: Springer, 2022. 4245–4251 [112] Ren Y B, Cao Y N, Fang F, Guo P, Lin Z, Ma W, et al. CLIO: Role-interactive multi-event head attention network for document-level event extraction. In: Proceedings of the 29th International Conference on Computational Linguistics. Gyeongju, Korea: Association for Computational Linguistics, 2022. 2504–2514 [113] Xu R X, Wang P Y, Liu T Y, Zeng S, Chang B B, Sui Z F. A two-stream AMR-enhanced model for document-level event argument extraction. In: Proceedings of the Conference of the North American Chapter of the Association for Computational Linguistics: Human Language Technologies. Seattle, USA: Association for Computational Linguistics, 2022. 5025–5036 [114] Yang Y Q, Guo Q P, Hu X K, Zhang Y, Qiu X P, Zhang Z. An AMR-based link prediction approach for document-level event argument extraction. In: Proceedings of the 61st Annual Meeting of the Association for Computational Linguistics. Toronto, Canada: Association for Computational Linguistics, 2023. 12876–12889 [115] Liu J, Liang C, Xu J N, Liu H Y, Zhao Z. Document-level event argument extraction with a chain reasoning paradigm. In: Proceedings of the 61st Annual Meeting of the Association for Computational Linguistics. Toronto, Canada: Association for Computational Linguistics, 2023. 9570–9583 [116] Liu W L, Cheng S H, Zeng D Y, QU H. Enhancing document-level event argument extraction with contextual clues and role relevance. In: Findings of the Association for Computational Linguistics: ACL. Toronto, Canada: Association for Computational Linguistics, 2023. 12908–12922 [117] Ren Y B, Cao Y N, Guo P, Fang F, Ma W, Lin Z. Retrieve-and-sample: Document-level event argument extraction via hybrid retrieval augmentation. In: Proceedings of the 61st Annual Meeting of the Association for Computational Linguistics. Toronto, Canada: Association for Computational Linguistics, 2023. 293–306 [118] Xu R X, Liu T Y, Li L, Chang B B. Document-level event extraction via heterogeneous graph-based interaction model with a tracker. In: Proceedings of the 59th Annual Meeting of the Association for Computational Linguistics and the 11th International Joint Conference on Natural Language Processing. Virtual Event: Association for Computational Linguistics, 2021. 3533–3546 [119] Liang Y, Jiang Z X, Yin D, Ren B. RAAT: Relation-augmented attention transformer for relation modeling in document-level event extraction. In: Proceedings of the Conference of the North American Chapter of the Association for Computational Linguistics: Human Language Technologies. Seattle, USA: Association for Computational Linguistics, 2022. 4985–4997 [120] Yang H, Sui D B, Chen Y B, Liu K, Zhao J, Wang T F. Document-level event extraction via parallel prediction networks. In: Proceedings of the 59th Annual Meeting of the Association for Computational Linguistics and the 11th International Joint Conference on Natural Language Processing. Virtual Event: Association for Computational Linguistics, 2021. 6298–6308 [121] Huang Y S, Jia W J. Exploring sentence community for document-level event extraction. In: Proceedings of Findings of the Association for Computational Linguistics: EMNLP. Punta Cana, Dominican Republic: Association for Computational Linguistics, 2021. 340–351 [122] Zhu T, Qu X Y, Chen W L, Wang Z F, Huai B X, Yuan N, et al. Efficient document-level event extraction via pseudo-trigger-aware pruned complete graph. In: Proceedings of the 31st International Joint Conference on Artificial Intelligence. Vienna, Austria: Springer, 2022. 4552–4558 [123] Wan Q Z, Wan C X, Xiao K L, Xiong H, Liu D X, Liu X P. Token-event-role structure-based multi-channel document-level event extraction. arXiv preprint arXiv: 2306.17733, 2023: 1–18 [124] Wan Q Z, Wan C X, Xiao K L, Liu D X, Li C L, Zheng B L, et al. Joint document-level event extraction via token-token bidirectional event completed graph. In: Proceedings of the 61st Annual Meeting of the Association for Computational Linguistics. Toronto, Canada: Association for Computational Linguistics, 2023. 10481–10492 [125] Wang X Y, Gui L, He Y L. Document-level multi-event extraction with event proxy nodes and hausdorff distance minimization. In: Proceedings of the 61st Annual Meeting of the Association for Computational Linguistics. Toronto, Canada: Association for Computational Linguistics, 2023. 10118–10133 [126] Araki J, Mitamura T. Open-domain event detection using distant supervision. In: Proceedings of the 27th International Conference on Computational Linguistics. Santa Fe, USA: Association for Computational Linguistics, 2018. 878–891 [127] Naik A, Rose C. Towards open domain event trigger identification using adversarial domain adaptation. In: Proceedings of the 58th Annual Meeting of the Association for Computational Linguistics. Virtual Event: Association for Computational Linguistics, 2020. 7618–7624 [128] Liu X, Huang H Y, Zhang Y. Open domain event extraction using neural latent variable models. In: Proceedings of the 57th Annual Meeting of the Association for Computational Linguistics. Florence, Italy: Association for Computational Linguistics, 2019. 2860–2871 [129] Ding X, Zhang Y, Liu T, Duan J W. Using structured events to predict stock price movement: An empirical investigation. In: Proceedings of the Conference on Empirical Methods in Natural Language Processing. Doha, Qatar: Association for Computational Linguistics, 2014. 1415–1425 [130] Mintz M, Bills S, Snow R, Jurafsky D. Distant supervision for relation extraction without labeled data. In: Proceedings of the Joint Conference of the 47th Annual Meeting of the ACL and the 4th International Joint Conference on Natural Language Processing of the AFNLP. Singapore: Association for Computational Linguistics, 2009. 1003–1011 [131] Muis A O, Otant N, Vyas N, Xu R C, Yang Y M, Mitamura T, et al. Low-resource cross-lingual event type detection via distant supervision with minimal effort. In: Proceedings of the 27th International Conference on Computational Linguistics. Santa Fe, USA: Association for Computational Linguistics, 2018. 70–82 [132] Liu J, Chen Y B, Liu K, Zhao J. Neural cross-lingual event detection with minimal parallel resources. In: Proceedings of the 2019 Conference on Empirical Methods in Natural Language Processing and the 9th International Joint Conference on Natural Language Processing. Hong Kong, China: Association for Computational Linguistics, 2019. 738–748 [133] Huang L F, Ji H. Semi-supervised new event type induction and event detection. In: Proceedings of the Conference on Empirical Methods in Natural Language Processing. Virtual Event: Association for Computational Linguistics, 2020. 718–724 [134] Zhang R H, Wei W, Mao X L, Fang R, Chen D Y. HCL-TAT: A hybrid contrastive learning method for few-shot event detection with task-adaptive threshold. In: Findings of the Association for Computational Linguistics: EMNLP. Abu Dhabi, United Arab Emirates: Association for Computational Linguistics, 2022. 1808–1819 [135] Zhao K L, Jin X L, Bai L, Guo J F, Cheng X Q. Knowledge-enhanced self-supervised prototypical network for few-shot event detection. In: Findings of the Association for Computational Linguistics: EMNLP. Abu Dhabi, United Arab Emirates: Association for Computational Linguistics, 2022. 6295–6304 [136] Yang X J, Lu Y J, Petzold L. Few-shot document-level event argument extraction. In: Proceedings of the 61st Annual Meeting of the Association for Computational Linguistics. Toronto, Canada: Association for Computational Linguistics, 2023. 8029–8046 [137] Zhou Y, Chen Y B, Zhao J, Wu J, Xu J X, Li J L. What the role is vs. What plays the role: Semi-supervised event argument extraction via dual question answering. In: Proceedings of the 35th AAAI Conference on Artificial Intelligence. Virtual Event: AAAI, 2021. 14638–14646 [138] Zhou J, Zhang Q, Chen Q, Zhang Q, He L, Huang X J. A multi-format transfer learning model for event argument extraction via variational information bottleneck. In: Proceedings of the 29th International Conference on Computational Linguistics. Gyeongju, Korea: Association for Computational Linguistics, 2022. 1990–2000 [139] Wu Q Y, Tan M K, Song H J, Chen J, Ng M K. ML-FOREST: A multi-label tree ensemble method for multi-label classification. IEEE Transactions on Knowledge and Data Engineering, 2016, 28(10): 2665–2680 doi: 10.1109/TKDE.2016.2581161 [140] Li Q Z, Zhang Q. A unified model for financial event classification, detection and summarization. In: Proceedings of the 29th International Joint Conference on Artificial Intelligence. Yokohama, Japan: Springer, 2020. 4668–4674 [141] Yu X Y, Rong W G, Liu J S, Zhou D Y, Ouyang Y X, Xiong Z. LSTM-based end-to-end framework for biomedical event extraction. IEEE/ACM Transactions on Computational Biology and Bioinformatics, 2019, 17(6): 2029–2039 [142] Huang K H, Yang M, Peng N Y. Biomedical event extraction with hierarchical knowledge graphs. In: Proceedings of the Conference on Empirical Methods in Natural Language Processing: Findings. Virtual Event: Association for Computational Linguistics, 2020. 1277–1285 [143] Ramponi A, Goot R V D, Lombardo R, Plank B. Biomedical event extraction as sequence labeling. In: Proceedings of the Conference on Empirical Methods in Natural Language Processing. Virtual Event: Association for Computational Linguistics, 2020. 5357–5367 [144] Fei H, Ren Y F, Ji D H. A tree-based neural network model for biomedical event trigger detection. Information Sciences, 2020, 512: 175–185 doi: 10.1016/j.ins.2019.09.075 [145] Bekoulis G, Deleu J, Demeeester T, Develder C. Joint entity recognition and relation extraction as a multi-head selection problem. Expert Systems with Applications, 2018, 114: 34–45 doi: 10.1016/j.eswa.2018.07.032 [146] 陈亦琦, 钱铁云, 李万理, 梁贻乐. 基于复合关系图卷积的属性网络嵌入方法. 计算机研究与发展, 2020, 57(8): 1674–1682 doi: 10.7544/issn1000-1239.2020.20200206Chen Yi-Qi, Qian Tie-Yun, Li Wan-Li, Liang Yi-Le. Exploiting composite relation graph convolution for attributed network embedding. Journal of Computer Research and Development, 2020, 57(8): 1674–1682 doi: 10.7544/issn1000-1239.2020.20200206 [147] El-allaly E D, Sarrouti M, En-Nahnahi N, Alaoui S O E. MTTLADE: A multi-task transfer learning-based method for adverse drug events extraction. Information Processing and Management, 2021, 58(3): Article No. 102473 doi: 10.1016/j.ipm.2020.102473 [148] Fan B, Fan W G, Smith C, Garner H S. Adverse drug event detection and extraction from open data: A deep learning approach. Information Processing and Management, 2020, 57(1): Article No. 102131 doi: 10.1016/j.ipm.2019.102131 [149] Li P F, Zhou G D, Zhu Q M, Hou L B. Employing compositional semantics and discourse consistency in Chinese event extraction. In: Proceedings of the Joint Conference on Empirical Methods in Natural Language Processing and Computational Natural Language Learning. Jeju Island, Korea: Association for Computational Linguistics, 2012. 1006–1016 [150] Wei S, Korostl I, Nothman J, Hachey B. English event detection with translated language features. In: Proceedings of the 55th Annual Meeting of the Association for Computational Linguistics. Vancouver, Canada: Association for Computational Linguistics, 2017. 293–298 [151] Lin H Y, Lu Y J, Han X P, Le S. Nugget proposal networks for Chinese event detection. In: Proceedings of the 56th Annual Meeting of the Association for Computational Linguistics. Melbourne, Australia: Association for Computational Linguistics, 2018. 1565–1574 [152] 李培峰, 周国栋, 朱巧明. 基于语义的中文事件触发词抽取联合模型. 软件学报, 2016, 27(2): 280–294 doi: 10.13328/j.cnki.jos.004833Li Pei-Feng, Zhou Guo-Dong, Zhu Qiao-Ming. Semantics-based joint model for Chinese event trigger extraction. Journal of Software, 2016, 27(2): 280–294 doi: 10.13328/j.cnki.jos.004833 -
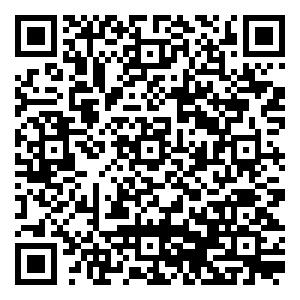
计量
- 文章访问数: 443
- HTML全文浏览量: 223
- 被引次数: 0