Data-driven Modeling and Predictive Algorithm for Complex Blast Furnace Ironmaking Process
-
摘要: 高炉炼铁过程的控制意味着控制高炉铁水温度及成份在指定的范围. 本文以高炉炉内热状态的重要指示剂---高炉铁水硅含量为研究对象, 针对机理建模难以准确预测、控制高炉铁水硅含量的发展变化, 利用数据驱动建模的思想, 建立了基于多元时间序列的高炉铁水硅含量数据驱动预测模型. 实例分析表明, 建立的数据驱动预测模型能够很好地预测高炉铁水硅含量, 连续预测167炉高炉铁水硅含量, 命中率高达83.23%, 预测均方根误差为0.07260. 这些指标均优于基于单一硅时间序列所建立的数据驱动模型, 对实际生产具有很好的指导作用.Abstract: Control of blast furnace ironmaking process means to control the temperature and compositions of blast furnace hot metal within specific bounds. For the silicon content in blast furnace hot metal as a targeted indicator of in-furnace thermal state, a data-driven based predictive model is constructed in this paper utilizing the information of multivariate time series measured from the blast finance system. Through an example, it is indicated that the constructed data-driven based predictive model has a good performance in predicting the silicon content in hot metal, with 83.23% percentage of target hitting and 0.07260 of the root mean square error of prediction when the size of predicted sample set is 167. These criteria are better than those achieved by data-driven predictive model based on univariate time series, implying that the constructed data-driven predictive model based on multivariate time series can act as an important tool to serve the production process.
-
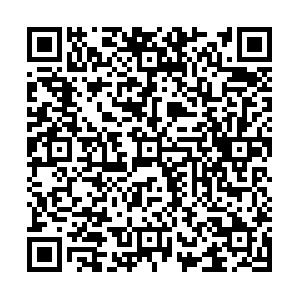
计量
- 文章访问数: 2266
- HTML全文浏览量: 104
- PDF下载量: 2447
- 被引次数: 0