A Bayesian Network Learning Algorithm Based on Independence Test and Ant Colony Optimization
-
摘要: 针对ACO-B蚁群算法学习贝叶斯网结构的不足, 提出了一种基于独立性测试和蚁群优化的改进算法. 新算法首先利用0阶独立性测试来限制侯选结构的搜索空间, 避免了蚁群的一些不必要的搜索, 然后融合解的全局评分增益和节点间局部的互信息, 给出了启发能力更强的启发函数来引导随机搜索. 实验结果表明, 新算法能够更有效地处理大规模数据, 且学习速度有较大改进.
-
关键词:
- 不确定性知识表示和推理 /
- Bayesian网络结构学习 /
- 蚁群算法 /
- 条件独立性测试
Abstract: To solve the drawbacks of the ant colony optimization for learning Bayesian networks (ACO-B), this paper proposes an improved algorithm based on the conditional independence test and ant colony optimization (I-ACO-B). First, the I-ACO-B uses order-0 independence tests to effectively restrict the space of candidate solutions, so that many unnecessary searches of ants can be avoided. And then, by combining the global score increase of a solution and local mutual information between nodes, a new heuristic function with better heuristic ability is given to induct the process of stochastic searches. The experimental results on the benchmark data sets show that the new algorithm is effective and efficient in large scale databases, and greatly enhances convergence speed compared to the original algorithm. -
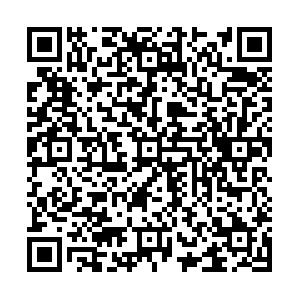
计量
- 文章访问数: 2074
- HTML全文浏览量: 94
- PDF下载量: 1486
- 被引次数: 0