-
摘要: In some sample based regression tasks, the observed samples are quite few or not informative enough. As a result, the conflict between the number of samples and the model complexity emerges, and the regression method will confront the dilemma whether to choose a complex model or not. Incorporating the prior knowledge is a potential solution for this dilemma. In this paper, a sort of the prior knowledge is investigated and a novel method to incorporate it into the kernel based regression scheme is proposed. The proposed prior knowledge based kernel regression (PKBKR) method includes two subproblems: representing the prior knowledge in the function space, and combining this representation and the training samples to obtain the regression function. A greedy algorithm for the representing step and a weighted loss function for the incorporation step are proposed. Finally, experiments are performed to validate the proposed PKBKR method, wherein the results show that the proposed method can achieve relatively high regression performance with appropriate model complexity, especially when the number of samples is small or the observation noise is large.Abstract: In some sample based regression tasks, the observed samples are quite few or not informative enough. As a result, the conflict between the number of samples and the model complexity emerges, and the regression method will confront the dilemma whether to choose a complex model or not. Incorporating the prior knowledge is a potential solution for this dilemma. In this paper, a sort of the prior knowledge is investigated and a novel method to incorporate it into the kernel based regression scheme is proposed. The proposed prior knowledge based kernel regression (PKBKR) method includes two subproblems: representing the prior knowledge in the function space, and combining this representation and the training samples to obtain the regression function. A greedy algorithm for the representing step and a weighted loss function for the incorporation step are proposed. Finally, experiments are performed to validate the proposed PKBKR method, wherein the results show that the proposed method can achieve relatively high regression performance with appropriate model complexity, especially when the number of samples is small or the observation noise is large.
-
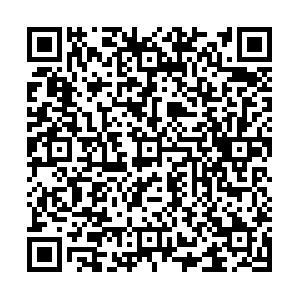
计量
- 文章访问数: 2623
- HTML全文浏览量: 81
- PDF下载量: 1698
- 被引次数: 0