-
摘要: 随着深度强化学习的研究与发展, 强化学习在博弈与优化决策、智能驾驶等现实问题中的应用也取得显著进展. 然而强化学习在智能体与环境的交互中存在人工设计奖励函数难的问题, 因此研究者提出了逆强化学习这一研究方向. 如何从专家演示中学习奖励函数和进行策略优化是一个新颖且重要的研究课题, 在人工智能领域具有十分重要的研究意义. 本文综合介绍了逆强化学习算法的最新进展, 首先介绍了逆强化学习在理论方面的新进展, 然后分析了逆强化学习面临的挑战以及未来的发展趋势, 最后讨论了逆强化学习的应用进展和应用前景.Abstract: With the research and development of deep reinforcement learning, the application of reinforcement learning in real-world problems such as game and optimization decision, and intelligent driving has also made significant progress. However, reinforcement learning has difficulty in manually designing the reward function in the interaction between an agent and its environment, so researchers have proposed the research direction of inverse reinforcement learning. How to learn reward functions from expert demonstrations and perform strategy optimization is a novel and important research topic with very important research implications in the field of artificial intelligence. This paper presents a comprehensive overview of the recent progress of inverse reinforcement learning algorithms. Firstly, new advances in the theory of inverse reinforcement learning are introduced, then the challenges faced by inverse reinforcement learning and the future development trends are analyzed, and finally the progress and application prospects of inverse reinforcement learning are discussed.
-
表 1 逆强化学习算法的研究历程
Table 1 Timeline of inverse reinforcement learning algorithm
逆强化学习算法 面临的挑战 解决的问题 作者 (年份) 有限和大状态空间的MDP/R问题 Ng等[9] (2000) 线性求解MDP/R问题 Abbeel等[11] (2004) 基于边际的逆强化学习 模糊歧义 策略的最大化结构与预测问题 Ratliff等[12] (2006) 复杂多维任务问题 Bogdanovic等[22] (2015) 现实任务的适用性问题 Hester等[23] (2018) 基于贝叶斯的逆强化学习 先验知识的选取难、计算复杂 结合先验知识和专家数据推导奖励的概率分布问题 Ramachandran等[21] (2007) 基于概率的逆强化学习 在复杂动态环境中适应性差 最大熵约束下的特征匹配问题 Ziebart等[13] (2008) 转移函数未知的MDP/R问题 Boularias等[14] (2011) 基于高斯过程的逆强化学习 计算复杂 奖励的非线性求解问题 Levine[19]等 (2011) 基于最大熵的深度逆强化学习 计算复杂、过拟合、专家
演示数据不平衡、有限从人类驾驶演示中学习复杂城市环境中奖励的问题 Wulfmeier等[15] (2016) 从数据中提取策略的对抗题性逆强化学习问题 Ho等[18] (2016) 多个奖励稀疏分散的线性可解非确定性MDP/R问题 Budhraja等[59] (2017) 自动驾驶车辆在交通中的规划问题 You等[38] (2019) 无模型积分逆RL的奖励问题 Lian等[70] (2021) 利用最大因果熵推断奖励函数的问题 Gleave等[94] (2022) 基于神经网络的逆强化学习 过拟合、不稳定 具有大规模高维状态空间的自动导航的IRL问题 Chen等[62] (2019) -
[1] 柴天佑. 工业人工智能发展方向. 自动化学报, 2020, 46(10): 2005-2012 doi: 10.16383/j.aas.c200796Chai Tian-You. Development directions of industrial artificial intelligence. Acta Automatica Sinica, 2020, 46(10): 2005-2012 doi: 10.16383/j.aas.c200796 [2] Dai X Y, Zhao C, Li X S, Wang X, Wang F Y. Traffic signal control using offline reinforcement learning. In: Proceedings of the China Automation Congress (CAC). Beijing, China: IEEE, 2021. 8090−8095 [3] Li J N, Ding J L, Chai T Y, Lewis F L. Nonzero-sum game reinforcement learning for performance optimization in large-Scale industrial processes. IEEE Transactions on Cybernetics, 2020, 50(9): 4132-4145 doi: 10.1109/TCYB.2019.2950262 [4] 赵冬斌, 邵坤, 朱圆恒, 李栋, 陈亚冉, 王海涛, 等. 深度强化学习综述: 兼论计算机围棋的发展. 控制理论与应用, 2016, 33(6): 701-717Zhao Dong-Bin, Shao Kun, Zhu Yuan-Heng, Li Dong, Chen Ya-Ran, Wang Hai-Tao, et al. Review of deep reinforcement learning and discussions on the development of computer Go. Control Theory & Applications, 2016, 33(6): 701-717 [5] Song T H, Li D Z, Yang W M, Hirasawa K. Recursive least-squares temporal difference with gradient correction. IEEE Transactions on Cybernetics, 2021, 51(8): 4251-4264 doi: 10.1109/TCYB.2019.2902342 [6] Bain M, Sammut C. A framework for Behavioural cloning. Machine Intelligence 15: Intelligent Agents, 1995: 103−129 [7] Couto G C K, Antonelo E A. Generative adversarial imitation learning for end-to-end autonomous driving on urban environments. In: Proceedings of the IEEE Symposium Series on Computational Intelligence (SSCI). Orlando, USA: IEEE, 2021. 1−7 [8] Samak T V, Samak C V, Kandhasamy S. Robust behavioral cloning for autonomous vehicles using end-to-end imitation learning. SAE International Journal of Connected and Automated Vehicles, 2021, 4(3): 279-295 [9] Ng A Y, Russell S J. Algorithms for inverse reinforcement learning. In: Proceedings of the 17th International Conference on Machine Learning (ICML). Stanford, USA: Morgan Kaufmann Publishers Inc, 2000. 663−670 [10] Imani M, Ghoreishi S F. Scalable inverse reinforcement learning through multifidelity Bayesian optimization. IEEE Transactions on Neural Networks and Learning Systems, 2022, 33(8): 4125-4132 doi: 10.1109/TNNLS.2021.3051012 [11] Abbeel P, Ng A Y. Apprenticeship learning via inverse reinforcement learning. In: Proceedings of the 21st International Conference on Machine Learning (ICML). Ban, Canada: ACM, 2004. 1−8 [12] Ratliff N D, Bagnell J A. Zinkevich M A. Maximum margin planning. In: Proceedings of the 23rd International Conference on Machine Learning (ICML). Pittsburgh, USA: ACM, 2006. 729−736 [13] Ziebart B D, Maas A, Bagnell J A, Dey A K. Maximum entropy inverse reinforcement learning. In: Proceedings of the 23rd AAAI Conference on Artificial Intelligence (AAAI). Chicago, USA: AAAI, 2008. 1433−1438 [14] Boularias A, Kober J, Peters J. Relative entropy inverse reinforcement learning. In: Proceedings of the 14th International Conference on Artificial Intelligence and Statistics (AISTATS). Fort Lauderdale, USA: 2011. 182−189 [15] Wulfmeier M, Wang D Z, Posner I. Watch this: Scalable cost-function learning for path planning in urban environments. In: Proceedings of the IEEE/RSJ International Conference on Intelligent Robots and Systems (IROS). Daejeon, Korea (South): IEEE, 2016. 2089−2095 [16] Guo H Y, Chen Q X, Xia Q, Kang C Q. Deep inverse reinforcement learning for objective function identification in bidding models. IEEE Transactions on Power Systems, 2021, 36(6): 5684-5696 doi: 10.1109/TPWRS.2021.3076296 [17] Shi YC, Jiu B, Yan J K, Liu H W, Li K. Data-driven simultaneous multibeam power allocation: When multiple targets tracking meets deep reinforcement learning. IEEE Systems Journal, 2021, 15(1): 1264-1274 doi: 10.1109/JSYST.2020.2984774 [18] Ho J, Ermon S. Generative adversarial imitation learning. In: Proceedings of the 30th International Conference on Neural Information Processing Systems (NIPS). Barcelona, Spain: Curran Associates Inc, 2016. 4572−4580 [19] Levine S, Popović Z, Koltun V. Nonlinear inverse reinforcement learning with Gaussian processes. In: Proceedings of the 24th International Conference on Neural Information Processing Systems (NIPS). Granada, Spain: Curran Associates Inc, 2011. 19−27 [20] Liu J H, Huang Z H, Xu X, Zhang X L, Sun S L, Li D Z. Multi-kernel online reinforcement learning for path tracking control of intelligent vehicles. IEEE Transactions on Systems, Man, and Cybernetics: Systems, 2021, 51(11): 6962-6975 doi: 10.1109/TSMC.2020.2966631 [21] Ramachandran D, Amir E. Bayesian inverse reinforcement learning. In: Proceedings of the 20th International Joint Conference on Artificial Intelligence (IJCAI). Hyderabad, India: Morgan Kaufmann, 2007. 2586−2591 [22] Bogdanovic M, Markovikj D, Denil M, de Freitas N. Deep apprenticeship learning for playing video games. In: Proceedings of the AAAI Workshop on Learning for General Competency in Video Games. Austin, USA: AAAI, 2015. 7−9 [23] Hester T, Vecerik M, Pietquin O, Lanctot M, Schaul T, Piot B, et al. Deep Q-learning from demonstrations. In: Proceedings of the 32nd AAAI Conference on Artificial Intelligence and 30th Innovative Applications of Artificial Intelligence Conference and 8th AAAI Symposium on Educational Advances in Artificial Intelligence. New Orleans, USA: AAAI, 2018. Article No. 394 [24] Nguyen H T, Garratt M, Bui L T, Abbass H. Apprenticeship learning for continuous state spaces and actions in a swarm-guidance shepherding task. In: Proceedings of the IEEE Symposium Series on Computational Intelligence (SSCI). Xiamen, China: IEEE, 2019. 102−109 [25] Hwang M, Jiang W C, Chen Y J. A critical state identification approach to inverse reinforcement learning for autonomous systems. International Journal of Machine Learning and Cybernetics, 2022, 13(4): 1409-1423 [26] 金卓军, 钱徽, 陈沈轶, 朱淼良. 基于回报函数逼近的学徒学习综述. 华中科技大学学报(自然科学版), 2008, 36(S1): 288-290, 294 doi: 10.13245/j.hust.2008.s1.081Jin Zhuo-Jun, Qian Hui, Chen Shen-Yi, Zhu Miao-Liang. Survey of apprenticeship learning based on reward function approximating. Journal of Huazhong University of Science & Technology (Nature Science Edition), 2008, 36(S1): 288-290, 294 doi: 10.13245/j.hust.2008.s1.081 [27] Levine S, Popović Z, Koltun V. Feature construction for inverse reinforcement learning. In: Proceedings of 23rh International Conference on Neural Information Processing Systems (NIPS). Vancouver, Canada: Curran Associates Inc, 2010. 1342−1350 [28] Pan W, Qu R P, Hwang K S, Lin H S. An Ensemble fuzzy approach for inverse reinforcement learning. International Journal of Fuzzy Systems, 2019, 21(1): 95-103 doi: 10.1007/s40815-018-0535-y [29] Lin J L, Hwang K S, Shi H B, Pan W. An ensemble method for inverse reinforcement learning. Information Sciences, 2020, 512: 518-532 doi: 10.1016/j.ins.2019.09.066 [30] Ratliff N, Bradley D, Bagnell J A, Chestnutt J. Boosting structured prediction for imitation learning. In: Proceedings of 19th International Conference on Neural Information Processing Systems (NIPS). Vancouver, Canada: MIT Press, 2006. 1153−1160 [31] Choi D, An T H, Ahn K, Choi J. Future trajectory prediction via RNN and maximum margin inverse reinforcement learning. In: Proceedings of the 17th IEEE International Conference on Machine Learning and Applications (ICMLA). Orlando, USA: IEEE, 2018. 125−130 [32] 高振海, 闫相同, 高菲. 基于逆向强化学习的纵向自动驾驶决策方法. 汽车工程, 2022, 44(7): 969-975 doi: 10.19562/j.chinasae.qcgc.2022.07.003Gao Zhen-Hai, Yan Xiang-Tong, Gao Fei. A decision-making method for longitudinal autonomous driving based on inverse reinforcement learning. Automotive Engineering, 2022, 44(7): 969-975 doi: 10.19562/j.chinasae.qcgc.2022.07.003 [33] Finn C, Levine S, Abbeel P. Guided cost learning: Deep inverse optimal control via policy optimization. In: Proceedings of the 33rd International Conference on International Conference on Machine Learning (ICML). New York, USA: JMLR.org, 2016. 49−58 [34] Fu J, Luo K, Levine S. Learning robust rewards with adversarial inverse reinforcement learning. In: Proceedings of 6th International Conference on Learning Representations (ICLR). Vancouver, Canada: Elsevier, 2018. 1−15 [35] Huang D A, Kitani K M. Action-reaction: Forecasting the dynamics of human interaction. In: Proceedings of the 13th European Conference on Computer Vision (ECCV). Zurich, Switzerland: Springer, 2014. 489−504 [36] Levine S, Koltun V. Continuous inverse optimal control with locally optimal examples. In: Proceedings of the 29th International Conference on Machine Learning (ICML). Edinburgh, Scotland: Omnipress, 2012. 475−482 [37] Aghasadeghi N, Bretl T. Maximum entropy inverse reinforcement learning in continuous state spaces with path integrals. In: Proceedings of the IEEE/RSJ International Conference on Intelligent Robots and Systems (IROS). San Francisco, USA: IEEE, 2011. 1561−1566 [38] You C X, Lu J B, Filev D, Tsiotras P. Advanced planning for autonomous vehicles using reinforcement learning and deep inverse reinforcement learning. Robotics and Autonomous Systems, 2019, 114: 1-18 doi: 10.1016/j.robot.2019.01.003 [39] Das N, Chattopadhyay A. Inverse reinforcement learning with constraint recovery. arXiv preprint arXiv: 2305.08130, 2023. [40] Krishnan S, Garg A, Liaw R, Miller L, Pokorny F T, Goldberg K. HIRL: Hierarchical inverse reinforcement learning for long-horizon tasks with delayed rewards. arXiv: 1604.06508, 2016. [41] Zhou Z Y, Bloem M, Bambos N. Infinite time horizon maximum causal entropy inverse reinforcement learning. IEEE Transactions on Automatic Control, 2018, 63(9): 2787-2802 doi: 10.1109/TAC.2017.2775960 [42] Wu Z, Sun L T, Zhan W, Yang C Y, Tomizuka M. Efficient sampling-based maximum entropy inverse reinforcement learning with application to autonomous driving. IEEE Robotics and Automation Letters, 2020, 5(4): 5355-5362 doi: 10.1109/LRA.2020.3005126 [43] Huang Z Y, Wu J D, Lv C. Driving behavior modeling using naturalistic human driving data with inverse reinforcement learning. IEEE Transactions on Intelligent Transportation Systems, 2022, 23(8): 10239-10251 doi: 10.1109/TITS.2021.3088935 [44] Song L, Li D Z, Xu X. Sparse online maximum entropy inverse reinforcement learning via proximal optimization and truncated gradient. Knowledge-Based Systems, 2022, 252: Article No. 109443 [45] Gleave A, Habryka O. Multi-task maximum causal entropy inverse reinforcement learning. arXiv: 1805.08882, 2018. [46] Zhang T, Liu Y, Hwang M, Hwang K S, Ma C Y, Cheng J. An end-to-end inverse reinforcement learning by a boosting approach with relative entropy. Information Sciences, 2020, 520: 1-14 [47] 吴少波, 傅启明, 陈建平, 吴宏杰, 陆悠. 基于相对熵的元逆强化学习方法. 计算机科学, 2021, 48(9): 257-263 doi: 10.11896/jsjkx.200700044Wu Shao-Bo, Fu Qi-Ming, Chen Jian-Ping, Wu Hong-Wei, Lu You. Meta-inverse reinforcement learning method based on relative entropy. Computer Science, 2021, 48(9): 257-263 doi: 10.11896/jsjkx.200700044 [48] Lin B Y, Cook D J. Analyzing sensor-based individual and population behavior patterns via inverse reinforcement learning. Sensors, 2020, 20(18): Article No. 5207 doi: 10.3390/s20185207 [49] Zhou W C, Li W C. A hierarchical Bayesian approach to inverse reinforcement learning with symbolic reward machines. In: Proceedings of the 39th International Conference on Machine Learning. Baltimore, USA: PMLR, 2022. 27159−27178 [50] Ezzeddine A, Mourad N, Araabi B N, Ahmadabadi M N. Combination of learning from non-optimal demonstrations and feedbacks using inverse reinforcement learning and Bayesian policy improvement. Expert Systems With Applications, 2018, 112: 331-341 [51] Ranchod P, Rosman B, Konidaris G. Nonparametric Bayesian reward segmentation for skill discovery using inverse reinforcement learning. In: Proceedings of the IEEE/RSJ International Conference on Intelligent Robots and Systems (IROS). Hamburg, Germany: IEEE, 2015. 471−477 [52] Choi J, Kim K E. Nonparametric Bayesian inverse reinforcement learning for multiple reward functions. In: Proceedings of the 25th International Conference on Neural Information Processing Systems. Lake Tahoe, USA: Curran Associates Inc, 2012. 305−313 [53] Okal B, Arras K O. Learning socially normative robot navigation behaviors with Bayesian inverse reinforcement learning. In: Proceedings of the IEEE International Conference on Robotics and Automation (ICRA). Stockholm, Sweden: IEEE, 2016. 2889−2895 [54] Trinh T, Brown D S. Autonomous assessment of demonstration sufficiency via bayesian inverse reinforcement learning. arXiv: 2211.15542, 2022. [55] Huang W H, Braghin F, Wang Z. Learning to drive via apprenticeship learning and deep reinforcement learning. In: Proceedings of the IEEE 31st International Conference on Tools with Artificial Intelligence (ICTAI). Portland, USA: IEEE, 2019. 1536−1540 [56] Markovikj D. Deep apprenticeship learning for playing games. arXiv preprint arXiv: 2205.07959, 2022. [57] Lee D, Srinivasan S, Doshi-Velez F. Truly batch apprenticeship learning with deep successor features. In: Proceedings of the 28th International Joint Conference on Artificial Intelligence (IJCAI). Macao, China: Morgan Kaufmann, 2019. 5909−5915 [58] Xia C, El Kamel A. Neural inverse reinforcement learning in autonomous navigation. Robotics and Autonomous Systems, 2016, 84: 1-14. [59] Budhraja K K, Oates T. Neuroevolution-based inverse reinforcement learning. In: Proceedings of the IEEE Congress on Evolutionary Computation (CEC). Donostia, Spain: IEEE, 2017. 67−76 [60] Memarian F, Xu Z, Wu B, Wen M, Topcu U. Active task-inference-guided deep inverse reinforcement learning. In: Proceedings of the 59th IEEE Conference on Decision and Control (CDC). Jeju, Korea (South): IEEE, 2020. 1932−1938 [61] Liu S, Jiang H, Chen S P, Ye J, He R Q, Sun Z Z. Integrating Dijkstra’s algorithm into deep inverse reinforcement learning for food delivery route planning. Transportation Research Part E: Logistics and Transportation Review, 2020, 142: Article No. 102070 doi: 10.1016/j.tre.2020.102070 [62] Chen X L, Cao L, Xu Z X, Lai J, Li C X. A study of continuous maximum entropy deep inverse reinforcement learning. Mathematical Problems in Engineering, 2019, 2019: Article No. 4834516 [63] Choi D, Min K, Choi J. Regularising neural networks for future trajectory prediction via inverse reinforcement learning framework. IET Computer Vision, 2020, 14(5): 192-200 doi: 10.1049/iet-cvi.2019.0546 [64] Wang Y, Wan S, Li Q, Niu Y, Ma F. Modeling crossing behaviors of E-Bikes at intersection with deep maximum entropy inverse reinforcement learning using drone-based video data. IEEE Transactions on Intelligent Transportation Systems, 2023, 24(6): 6350−6361 [65] Fahad M, Chen Z, Guo Y. Learning how pedestrians navigate: A deep inverse reinforcement learning approach. In: Proceedings of the IEEE/RSJ International Conference on Intelligent Robots and Systems (IROS). Madrid, Spain: IEEE, 2018. 819−826 [66] Zhou Y, Fu R, Wang C. Learning the car-following behavior of drivers using maximum entropy deep inverse reinforcement learning. Journal of Advanced Transportation, 2020, 2020: Article No. 4752651 [67] Song L, Li D Z, Wang X, Xu X. AdaBoost maximum entropy deep inverse reinforcement learning with truncated gradient. Information Sciences, 2022, 602: 328-350 doi: 10.1016/j.ins.2022.04.017 [68] Wang P, Liu D P, Chen J Y, Li H H, Chan C Y. Decision making for autonomous driving via augmented adversarial inverse reinforcement learning. In: Proceedings of the IEEE International Conference on Robotics and Automation (ICRA). Xi’an, China: IEEE, 2021. 1036−1042 [69] Sun J K, Yu L T, Dong P Q, Lu B, Zhou B L. Adversarial inverse reinforcement learning with self-attention dynamics model. IEEE Robotics and Automation Letters, 2021, 6(2): 1880-1886 doi: 10.1109/LRA.2021.3061397 [70] Lian B S, Xue W Q, Lewis F L, Chai T Y. Inverse reinforcement learning for adversarial apprentice games. IEEE Transactions on Neural Networks and Learning Systems, DOI: 10.1109/TNNLS.2021.3114612 [71] Jin Z J, Qian H, Zhu M L. Gaussian processes in inverse reinforcement learning. In: Proceedings of the International Conference on Machine Learning and Cybernetics (ICMLC). Qingdao, China: IEEE, 2010. 225−230 [72] Li D C, He Y Q, Fu F. Nonlinear inverse reinforcement learning with mutual information and Gaussian process. In: Proceedings of the IEEE International Conference on Robotics and Biomimetics (ROBIO). Bali, Indonesia: IEEE, 2014. 1445−1450 [73] Michini B, Walsh T J, Agha-Mohammadi A A, How J P. Bayesian nonparametric reward learning from demonstration. IEEE Transactions on Robotics, 2015, 31(2): 369-386 doi: 10.1109/TRO.2015.2405593 [74] Sun L T, Zhan W, Tomizuka M. Probabilistic prediction of interactive driving behavior via hierarchical inverse reinforcement learning. In: Proceedings of the 21st International Conference on Intelligent Transportation Systems (ITSC). Maui, USA: IEEE, 2018. 2111−2117 [75] Rosbach S, Li X, Großjohann S, Homoceanu S, Roth S. Planning on the fast lane: Learning to interact using attention mechanisms in path integral inverse reinforcement learning. In: Proceedings of the IEEE/RSJ International Conference on Intelligent Robots and Systems (IROS). Las Vegas, USA: IEEE, 2020. 5187−5193 [76] Rosbach S, James V, Großjohann S, Homoceanu S, Roth S. Driving with style: Inverse reinforcement learning in general-purpose planning for automated driving. In: Proceedings of the IEEE/RSJ International Conference on Intelligent Robots and Systems (IROS). Macau, China: IEEE, 2019. 2658−2665 [77] Fernando T, Denman S, Sridharan S, Fookes C. Deep inverse reinforcement learning for behavior prediction in autonomous driving: Accurate forecasts of vehicle motion. IEEE Signal Processing Magazine, 2021, 38(1): 87-96 doi: 10.1109/MSP.2020.2988287 [78] Fernando T, Denman S, Sridharan S, Fookes C. Neighbourhood context embeddings in deep inverse reinforcement learning for predicting pedestrian motion over long time horizons. In: Proceedings of the IEEE/CVF International Conference on Computer Vision Workshop (ICCVW). Seoul, Korea (South): IEEE, 2019. 1179−1187 [79] Kalweit G, Huegle M, Werling M, Boedecker J. Deep inverse Q-learning with constraints. In: Proceedings of the 34th International Conference on Neural Information Processing Systems. Vancouver, Canada: Curran Associates Inc, 2020. Article No. 1198 [80] Zhu Z Y, Li N, Sun R Y, Xu D H, Zhao H J. Off-road autonomous vehicles traversability analysis and trajectory planning based on deep inverse reinforcement learning. In: Proceedings of the IEEE Intelligent Vehicles Symposium (IV). Las Vegas, USA: IEEE, 2020. 971−977 [81] Fang P Y, Yu Z P, Xiong L, Fu Z Q, Li Z R, Zeng D Q. A maximum entropy inverse reinforcement learning algorithm for automatic parking. In: Proceedings of the 5th CAA International Conference on Vehicular Control and Intelligence (CVCI). Tianjin, China: IEEE, 2021. 1−6 [82] Pan X, Ohn-Bar E, Rhinehart N, Xu Y, Shen Y L, Kitani K M. Human-interactive subgoal supervision for efficient inverse reinforcement learning. In: Proceedings of the 17th International Conference on Autonomous Agents and MultiAgent Systems. Stockholm, Sweden: International Foundation for Autonomous Agents and Multiagent Systems, 2018. [83] Peters J, Mülling K, Altün Y, Peters J. Mulling K, Altun Y. Relative entropy policy search. In: Proceedings of 24th AAAI Conference on Artificial Intelligence (AAAI). Atlanta, Georgia: AAAI, 2010. 1607−1612 [84] Singh A, Yang L, Hartikainen K, Finn C, Levine S. End-to-end robotic reinforcement learning without reward engineering. In: Proceedings of the Robotics: Science and Systems. Freiburg im Breisgau, Germany: the MIT Press, 2019. [85] Wang H, Liu X F, Zhou X. Autonomous UAV interception via augmented adversarial inverse reinforcement learning. In: Proceedings of the International Conference on Autonomous Unmanned Systems (ICAUS). Changsha, China: Springer, 2022. 2073−2084 [86] Choi S, Kim S, Kim H J. Inverse reinforcement learning control for trajectory tracking of a multirotor UAV. International Journal of Control, Automation and Systems, 2017, 15(4): 1826-1834 doi: 10.1007/s12555-015-0483-3 [87] Nguyen H T, Garratt M, Bui L T, Abbass H. Apprenticeship bootstrapping: Inverse reinforcement learning in a multi-skill UAV-UGV coordination task. In: Proceedings of the 17th International Conference on Autonomous Agents and MultiAgent Systems (AAMAS). Stockholm, Sweden: International Foundation for Autonomous Agents and Multiagent Systems, 2018. 2204−2206 [88] Sun W, Yan D S, Huang J, Sun C H. Small-scale moving target detection in aerial image by deep inverse reinforcement learning. Soft Computing, 2020, 24(8): 5897-5908 doi: 10.1007/s00500-019-04404-6 [89] Pattanayak K, Krishnamurthy V, Berry C. Meta-cognition. An inverse-inverse reinforcement learning approach for cognitive radars. In: Proceedings of the 25th International Conference on Information Fusion (FUSION). Linköping, Sweden: IEEE, 2022. 1−8 [90] Kormushev P, Calinon S, Saegusa R, Metta G. Learning the skill of archery by a humanoid robot iCub. In: Proceedings of the 10th IEEE-RAS International Conference on Humanoid Robots. Nashville, USA: IEEE, 2010. 417−423 [91] Koller D, Milch B. Multi-agent influence diagrams for representing and solving games. Games and Economic Behavior, 2003, 45(1): 181-221 doi: 10.1016/S0899-8256(02)00544-4 [92] Syed U, Schapire R E. A Game-theoretic approach to apprenticeship learning. In: Proceedings of 22nd Conference on Neural Information Processing Systems (NIPS). Vancouver, Canada: Curran Associates Inc, 2007. 1449−1456 [93] Halperin I, Liu J Y, Zhang X. Combining Reinforcement Learning and Inverse Reinforcement Learning for Asset Allocation Recommendations. arXiv: 2201.01874, 2022. [94] Gleave A, Toyer S. A primer on maximum causal entropy inverse reinforcement learning. arXiv: 2203.11409, 2022. [95] Adams S, Cody T, Beling P A. A survey of inverse reinforcement learning. Artificial Intelligence Review, 2022, 55(6): 4307-4346 doi: 10.1007/s10462-021-10108-x [96] Li X J, Liu H S, Dong M H. A general framework of motion planning for redundant robot manipulator based on deep reinforcement learning. IEEE Transactions on Industrial Informatics, 2022, 18(8): 5253-5263 doi: 10.1109/TII.2021.3125447 [97] Jeon W, Su C Y, Barde P, Doan T, Nowrouzezahrai D, Pineau J. Regularized inverse reinforcement learning. In: Proceedings of 9th International Conference on Learning Representations (ICLR). Vienna, Austria: Ithaca, 2021. 1−26 [98] Krishnamurthy V, Angley D, Evans R, Moran B. Identifying cognitive radars-inverse reinforcement learning using revealed preferences. IEEE Transactions on Signal Processing, 2020, 68: 4529-4542 doi: 10.1109/TSP.2020.3013516 [99] 陈建平, 陈其强, 傅启明, 高振, 吴宏杰, 陆悠. 基于生成对抗网络的最大熵逆强化学习. 计算机工程与应用, 2019, 55(22): 119-126 doi: 10.3778/j.issn.1002-8331.1904-0238Chen Jian-Ping, Chen Qi-Qiang, Fu Qi-Ming, Gao Zhen, Wu Hong-Jie, Lu You. Maximum entropy inverse reinforcement learning based on generative adversarial networks. Computer Engineering and Applications, 2019, 55(22): 119-126 doi: 10.3778/j.issn.1002-8331.1904-0238 [100] Gruver N, Song J M, Kochenderfer M J, Ermon S. Multi-agent adversarial inverse reinforcement learning with latent variables. In: Proceedings of the 19th International Conference on Autonomous Agents and MultiAgent Systems (AAMAS). Auckland, New Zealand: International Foundation for Autonomous Agents and Multiagent Systems, 2020. 1855−1857 [101] Giwa B H, Lee C G. A marginal log-likelihood approach for the estimation of discount factors of multiple experts in inverse reinforcement learning. In: Proceedings of the IEEE/RSJ International Conference on Intelligent Robots and Systems (IROS). Prague, Czech Republic: IEEE, 2021. 7786−7791 [102] Ghosh S, Srivastava S. Mapping language to programs using multiple reward components with inverse reinforcement learning. In: Proceedings of the Findings of the Association for Computational Linguistics. Punta Cana, Dominican Republic: ACL, 2021. 1449−1462 [103] Gronauer S, Diepold K. Multi-agent deep reinforcement learning: A survey. Artificial Intelligence Review, 2021, 55(2): 895-943 [104] Bergerson S. Multi-agent inverse reinforcement learning: Suboptimal demonstrations and alternative solution concepts. arXiv: 2109.01178, 2021. [105] Zhao J C. Safety-aware multi-agent apprenticeship learning. arXiv: 2201.08111, 2022. [106] Hwang R, Lee H, Hwang H J. Option compatible reward inverse reinforcement learning. Pattern Recognition Letters, 2022, 154: 83-89 doi: 10.1016/j.patrec.2022.01.016 -
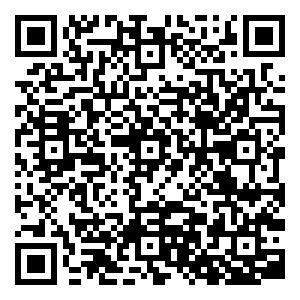
计量
- 文章访问数: 1593
- HTML全文浏览量: 897
- 被引次数: 0