Research Development in Theory and Application of Human Simulated Intelligent Control
-
摘要: 仿人智能控制是现代智能控制理论之一, 利用分层递阶的控制结构与多控制模态为强非线性、大迟滞、难建模问题提供了切实可行的解决方案, 近些年来发展迅速并且得到学术界的持续关注, 但缺乏对该理论研究进展系统性的总结. 本文通过系统的梳理仿人智能控制的理论基础和发展脉络, 将其划分为三代控制模型, 分别从每一代控制模型的算法描述、研究进展与应用进展三个角度进行综述, 同时, 结合当前的研究进展讨论仿人智能控制在控制模型、结构功能、参数校正方面进一步研究的方向.Abstract: Human simulated intelligent control is one of the modern intelligent control theories. The hierarchical control structure and multi-control modes are used to solve the problems of strong nonlinearity, large hysteresis and difficult modeling. In recent years, it has developed rapidly and received continuous attention from the academic community, but it lacks a systematic summary of the research progress in theory. This paper systematically sorts out the theoretical basis and development context of human simulated intelligent control, divides it into three generations of control models, and summarizes them from three perspectives: algorithm description, research progress and application progress of each generation of control models. In addition, considering the research progress, it also discusses the direction of further research on human simulated intelligent control in control models, structural functions, and parameter correction.
-
表 1 三代仿人智能控制关系表
Table 1 Relation table of three generations of human simulated intelligent control
控制模型 联系与发展改进 提出时间 服务对象 原型算法 用极值采样保持替代积分器, 能够很好的兼顾稳定性、快速性和精确性. 1979 温度控制、PH控制等 仿人智能控制(HSIC) 以原型算法为核心思想, 建立分层递阶的信息处理与决策机构, 基于特征模型在线特征辨识和特征记忆, 构建能够定性决策与定量控制的多模态集, 采用启发式推理的规则集. 1990 倒立摆、工业控制等具有强非线性、欠驱动特性的控制对象 基于动觉智能图式的仿人智能控制(SMIS-HSIC) 以第二代仿人智能控制为基础, 融合图式理论, 提升控制器的控制效果与智能程度, 赋予控制器自适应自学习能力, 加强控制器分布式、并行执行的特点. 2003 多级倒立摆、机器人等更复杂难控的对象 -
[1] 辛斌, 陈杰, 彭志红. 智能优化控制: 概述与展望. 自动化学报, 2013, 39(11): 1831−1848 doi: 10.3724/SP.J.1004.2013.01831Xin Bin, Chen Jie, Peng Zhi-Hong. Intelligent optimized control: overview and prospect. Acta Automatica Sinica, 2013, 39(11): 1831−1848 doi: 10.3724/SP.J.1004.2013.01831 [2] 周其鉴, 柏建国, 吕炳朝, 张兴华. 增益适应式非线性调节. 重庆大学学报(自然科学版), 1980, 04: 36−68Zhou Qi-Jian, Bai Jian-Guo, Lv Bing-Chao, Zhang Xing-Hua. A variable gain type of nonlinear adaptive controller. Journal of Chongqing University, 1980, 04: 36−68 [3] Zhou Qi-Jian, Bai Jian-Guo. An intelligent controller of novel design. In: Proceeding of a Multinational Instrumentation Conference. Shanghai, China: United States, 1983.127−149. [4] 李祖枢, 徐鸣, 周其鉴. 一种新型的仿人智能控制器(SHIC). 自动化学报, 1990, 06: 503−509Li Zu-Shu, Xu Ming, Zhou Qi-Jian. A novel simulating of human intelligent controller (SHIC). Acta Automatica Sinica, 1990, 06: 503−509 [5] 涂亚庆, 李祖枢, 黄尚廉. 仿人智能控制(SHIC)进展. 光学精密工程, 1993, 06: 40−44Tu Ya-Qing, Li Zu-Shu, Huang Shang-Lian. The progression of simulating human intelligent control. Optics and Precision Engineering, 1993, 06: 40−44 [6] 涂亚庆, 李祖枢. 一种新型的仿人智能控制器的设计方法. 自动化学报, 1994, 05: 616−621Tu Ya-Qing, Li Zu-Shu. Design method for a novel human simulating intelligent controller. Acta Automatica Sinica, 1994, 05: 616−621 [7] 李祖枢, 涂亚庆. 仿人智能控制. 北京: 国防工业出版社, 2003.Li Zu-Shu, Tu Ya-Qing. Human Simulated Intelligent Control Beijing: National Defense Industry Press, 2003. [8] 蔡自兴, 余伶俐, 肖晓明. 智能控制原理与应用. 北京: 清华大学出版社, 2014.Cai Zi-Xing, Yu Ling-Li, Xiao Xiao-Ming.Principles and Applications of Intelligent Control . Beijing: Tsinghua University Press, 2014. [9] 蔡自兴. 中国智能控制40年. 科技导报, 2018, 17: 23−39Cai Zi-Xing. 40 Years of intelligent control in China. Science & Technology Review, 2018, 17: 23−39 [10] Luo Zhong, Liu Hong-Yi, Wang Fei, Xu Li-Xia. Overview of human-simulated intelligent control method and its application. International Journal of Modelling, Identification and Control, 2010, 1-2(9): 161−167 [11] 陈民铀, 周其鉴. PH过程的智能控制. 信息与控制, 1987, 02: 1−6Chen Min-You, Zhou Qi-Jian. Intelligent control of PH process. Information & Control, 1987, 02: 1−6 [12] 杨飚, 张曾科. 仿人智能控制器的动态特性参数整定方法. 信息与控制, 2004, 06: 670−673 doi: 10.3969/j.issn.1002-0411.2004.06.007Yang Yan, Zhang Zeng-Ke. Dynamic characteristic parameter setting method for human-simulated intelligent controller. Information and Control, 2004, 06: 670−673 doi: 10.3969/j.issn.1002-0411.2004.06.007 [13] 刘军, 胡章军. 一种仿人智能控制参数整定方法. 控制工程, 2006, S1: 91−93 doi: 10.3969/j.issn.1671-7848.2006.01.025Liu Jun, Hu Zhang-Jun. Parameter setting method for human-simulated intelligent controller. Control Engineering of China, 2006, S1: 91−93 doi: 10.3969/j.issn.1671-7848.2006.01.025 [14] Wang Pei-Jin. Improvement of the prototype algorithm of human simulated intelligent control. In: Proceedings of International Conference on Machine Learning & Cybernetics. Kunming, China: IEEE, 2008.1998−2003. [15] 罗晓平, 黄海. 智能桁架结构振动的仿人智能控制. 振动与冲击, 2006, 25(06): 92−96 doi: 10.3969/j.issn.1000-3835.2006.06.023Luo Xiao-Ping. Human-simulated intelligent control for intelligent truss structures. Journal of Vibration and Shock, 2006, 25(06): 92−96 doi: 10.3969/j.issn.1000-3835.2006.06.023 [16] 白美卿, 高富强. 仿人智能温度控制器. 仪器仪表学报, 1988, 01: 85−89Bai Mei-Qin, Gao Fu-Qiang. Temperature-controller using an algorithm of intelligent control simulating humen behavior. Chinese Journal of Scientific Instrument, 1988, 01: 85−89 [17] 王昌银, 王应建, 林建亚. 机器人电液伺服关节的仿人智能控制实践. 机器人, 1990, 05: 25−29Wang Chang-Yin, Wang Ying-Jian, Lin Jian-Ya. Intelligent control of electro-hydraulic servo system. Robot, 1990, 05: 25−29 [18] 张虹, 陈淑羚, 张兵. 仿人智能控制器的模糊自整定. 内蒙古工业大学学报(自然科学版), 1999, 03: 201−205Zhang Hong, Chen Shu-Ling, Zhang Bing. A Self-tuning approach of HSIC based on fuzzy in ference. Journal of Innermongolia Polytechnic University, 1999, 03: 201−205 [19] Li Zu-Shu, Liang Dong-Wu, Chen Gui-Qiang. Fuzzy self-tuning of parameters online for human simulated intelligent controller. In: Proceedings of the IEEE International Conference on Systems, Man and Cybernetics. Montréal, Canada: IEEE, 2007.2402−2407. [20] Tian Jian-Yan, Zhang Guan-Yu, Wu Jin, Li Wen-Ting. The fuzzy human-simulated intelligent control for hot-rolling strip width. In: Proceedings of Intelligent Control & Automation. Beijing, China: IEEE, 2012.270−274. [21] Chen Gui-Qiang, Tang Lin-Jian, Li Zu-Shu. Parameter optimisation of human-simulated intelligent controller for a cart-double pendulum system. International Journal of Modelling, Identification and Control, 2010, 10(3/4): 194−201 doi: 10.1504/IJMIC.2010.034571 [22] 雷建和, 万斌, 刘明, 张栋. 基于粒子群算法的四旋翼仿人智能控制器设计. 计算机仿真, 2018, 35(01): 27−32 doi: 10.3969/j.issn.1006-9348.2018.01.006Lei Jian-He, Wan Bin, Liu Ming, Zhang Dong. The design of four-rotor human-simulated intelligent controller based on particle swarm optimization algorithm. Computer Simulation, 2018, 35(01): 27−32 doi: 10.3969/j.issn.1006-9348.2018.01.006 [23] 张贯宇, 田建艳, 张维杰, 邱华东, 张婵爱. 基于模糊神经网络参数整定的仿人智能控制. 传感器与微系统, 2013, 10: 30−33 doi: 10.3969/j.issn.1000-9787.2013.06.009Zhang Guan-Yu, Tian Jian-Yan, Zhang Wei-Jie, Qiu Hua-Dong, Zhang Chan-Ai. Human-simulated intelligent control based on parameters correcting by fuzzy neural network. Transducer and Microsystem Technologies, 2013, 10: 30−33 doi: 10.3969/j.issn.1000-9787.2013.06.009 [24] Xiao Qian-Jun, Wu Qian, Liu Bu-Qing. Optimization tuning model of control parameter based on artificial immune principle in human simulated intelligent controller. International Journal of Control and Automation, 2016, 9(8): 21−30 doi: 10.14257/ijca.2016.9.8.03 [25] 雷正桥. 仿人智能控制器控制参数的免疫优化方法. 辽宁工程技术大学学报(自然科学版), 2016, 35(01): 101−104Lei Zheng-Qiao. Method of immune optimization for control parameter in human simulated intelligent controller. Journal of Liaoning Technical University(Natural Science), 2016, 35(01): 101−104 [26] 盖晓华, 刘叔军, 张南纶. 一种模糊HSIC特征模型新算法的分析与仿真. 信息与控制, 2009, 38(02): 239−244 doi: 10.3969/j.issn.1002-0411.2009.02.020Gai Xiao-Hua, Liu Shu-Jun, Zhang Nan-Lun. Analysis and simulation on a new algorithm of characteristic model of fuzzy HSIC. Information & Control, 2009, 38(02): 239−244 doi: 10.3969/j.issn.1002-0411.2009.02.020 [27] 翟天嵩, 刘叔军, 张南纶. Robocup中型组足球机器人的改进模糊HSIC运动控制. 信息与控制, 2011, 40(04): 537−541Zhai Tian-Song, Liu Shu-Jun, Zhang Nan-Lun. An improved fuzzy-HSIC based motion control for robocup middle-sized soccer robot. Information and Control, 2011, 40(04): 537−541 [28] Zhang Feng-Rui, Huo Da-Yong. Research of human-simulated intelligent controller in industrial control. In: Proceedings of Second International Workshop on Education Technology & Computer Science. Wuhan, China: IEEE, 2010.216−219. [29] 祁虔. 自校正仿人智能控制器及其在等摆长倒立摆系统中的应用[博士学位论文], 重庆大学, 2017Qi Qian. Adaptive human simulated intelligent controller and its application in inverted pendulum system with uniform rods[Ph. D. dissertation], Chongqing University, 2017. [30] 王牛. 基于动觉智能图式足球机器人运动控制[博士学位论文], 重庆大学, 2008Wang Niu. Research of soccer robot human simulated intelligence motion control based on sensory-motor intelligence schema[Ph. D. dissertation], Chongqing University, 2008. [31] Zhang Qi, Wang Niu, Li Zu-Shu. Point stabilization control for two-wheel robot with online parameters settings. In: Proceedings of Intelligent Control & Automation. Taipei, China: IEEE, 2011.176−181. [32] Ran Song, Wang Niu, Pu Hua, Yin Chang-Wei, Wang Te-Te, Wang Teng-Fei. Adaptive point stabilization control of two-wheel robot with parameter uncertainties based on human-simulated intelligent backstepping method. In: Proceedings of The 37th China Control Conference. Wuhan, China: IEEE, 2018.3915−3920. [33] 陈晓龙, 章云, 杨玲玲. 基于确定有穷自动机描述的仿人智能控制记忆. 微电子学与计算机, 2008, 05: 88−90+94Chen Xiao-Long, Zhang Yun, Yang Ling-Ling. Memory of HSIC based on the describing about deterministic finite automata. Microelectronics & Computer, 2008, 05: 88−90+94 [34] Liu Yu-Cheng, Liu Yu-Bin. Intelligent integration between human simulated intelligence and expert control technology for the combustion process of gas heating furnace. Mathematical Problems in Engineering, 2014, 2014: 1−6 [35] 董小闵, 余淼, 陈伟民. 磁流变悬架的瞬态动力学控制研究. 振动工程学报, 2008, 21(02): 167−172 doi: 10.3969/j.issn.1004-4523.2008.02.012Dong Xiao-Min, Yu Miao, Chen Wei-Min. Control for transient dynamics of magneto-rheological suspension. Journal of Vibration Engineering, 2008, 21(02): 167−172 doi: 10.3969/j.issn.1004-4523.2008.02.012 [36] Yu Miao, Dong Xiao-Min, Choi S. B., Liao Chang-Rong. Human simulated intelligent control of vehicle suspension system with mr dampers. Journal of Sound and Vibration, 2009, 319(3-5): 753−767 [37] Xu Ai-Dong, Li Chuan-Qing, Chen Yan-Jun, Liu Guang-Sheng, Han Li. Model-free adaptive with human-simulated intelligent control and its application in super-heated steam temperature system. In: Proceedings of the 26th Chinese Control Conference. Hunan, China: IEEE, 2007.725−728. [38] Guo Cheng, Du Feng, Li Jin-Yu, Ren Jia, Zhang Yu, Zhou Hui. Networked control systems based on human-simulated intelligent and fuzzy control. In: Proceedings of World Automation Congress Proceedings. Puerto Vallarta, Mexico: IEEE, 2012.1−4. [39] 周彦, 何小阳, 王冬丽. 时变大滞后过程的专家模糊控制设计与仿真. 系统仿真学报, 2006, 10: 2786−2789 doi: 10.3969/j.issn.1004-731X.2006.10.023Zhou Yan, He Xiao-Yang, Wang Dong-Li. Design and simulation of expert fuzzy control for time-varying processes with large time delay. Journal of System Simulation, 2006, 10: 2786−2789 doi: 10.3969/j.issn.1004-731X.2006.10.023 [40] Lin Hai-Jun, Teng Zhao-Sheng, Chen Tao, Yang Sheng-Jie, Zhang Hong-Chuan, Chi Hai. Improved fuzzy control method for temperature in water tank of intelligent viscometer. In: Proceedings of IEEE International Conference on Information & Automation. Changsha, China: IEEE, 2008.1106−1110. [41] Qi Qian, Li Zu-Shu, Tan Zhi, Dan Yuan-Hong, Lin Xiao. Adaptive human-simulated intelligent controller and its application in double inverted pendulum. In: Proceedings of Intelligent Control & Automation. Jinan, China: IEEE, 2010.1063−1066. [42] Liu Cui-Ling, Sun Xiao-Rong, Chen Jian-Ming. Application of human-simulating intelligent control in heating the super-high pressure kettle. In: Proceedings of World Congress on Intelligent Control & Automation. Beijing, China: IEEE, 2008.7070−7073. [43] 李祖枢. 力矩受限单摆的摆起倒立控制——仿人智能控制在非线性系统中的应用. 控制理论与应用, 1999, 02: 225−229 doi: 10.3969/j.issn.1000-8152.1999.02.016Li Zu-Shu. The control for swinging up of a pendulum under limited torquen application of human simulated intelligent control in nonlinear system. Control Theory & Applications, 1999, 02: 225−229 doi: 10.3969/j.issn.1000-8152.1999.02.016 [44] 雷李, 李祖枢, 王牛. 基于仿人智能控制的足球机器人底层运动研究. 哈尔滨工业大学学报, 2004, 07: 978−980 doi: 10.3321/j.issn:0367-6234.2004.07.044Lei Li, Li Zu-Shu, Wang Niu. Study of robot soccer's basic motion based on HSIC. Journal of Harbin Institute of Technology, 2004, 07: 978−980 doi: 10.3321/j.issn:0367-6234.2004.07.044 [45] Luo Zhong, Liu Hong-Yi, Wang Fei, Li Li-Na, Yang Jiang. The human-simulation intelligent control of contact force based on the detection of ultrasonic sensors. In: Proceedings of IEEE International Conference on Robotics & Biomimetics. Sanya, China: IEEE, 2007.1664−1668. [46] Luo Zhong, Liu Hong-Yi, Wang Fei. Error analysis of robot detecting system with laser sensors in unknown environment. International Journal of Modelling, Identification and Control, 2011, 12(1/2): 160−165 doi: 10.1504/IJMIC.2011.037845 [47] Li Tie-Jun, Chen Gui-Qiang, Shao Gui-Fang. Action control of soccer robots based on simulated human intelligence. International Journal of Automation and Computing, 2010, 7(1): 55−63 doi: 10.1007/s11633-010-0055-1 [48] 敬成林, 朱晓铭. 仿人预测控制在双足机器人步行控制中的应用. 传感器与微系统, 2014, 33(03): 157−160 doi: 10.3969/j.issn.1000-9787.2014.03.045Jing Cheng-Lin, Zhu Xiao-Ming. Application of human-simulated predictive control in walking control of biped robot. Transducer and Microsystem Technologies, 2014, 33(03): 157−160 doi: 10.3969/j.issn.1000-9787.2014.03.045 [49] 陈情, 薛方正. 工业机器人的仿人智能控制. 重庆理工大学学报(自然科学), 2012, 26(07): 42−49Chen Qing, Xue Fang-Zheng. Human-simulated intelligent control for manipulator. Journal of Chongqing University of Technology (Natural Science), 2012, 26(07): 42−49 [50] Liu Rong-Sheng, Gao Ying-Jie, Yang Yu-Lin, Liu Yan-Long. Human simulated intelligent control with double-direction dead-zone compensation for joint motion control of a large-sized boom system. Journal of Control Science and Engineering, 2015, 2015: 1−7 [51] 刘成军, 薛方正, 李祖枢, 钟灶生, 敬成林. 基于分时段模型的无刷直流电机仿人智能控制. 系统仿真学报, 2011, 23(06): 1098−1103Liu Cheng-Jun, Xue Fang-Zheng, Li Zu-Shu, Zhong Zao-Sheng, Jing Cheng-Lin. Human simulated intelligent control of brushless dc motor based on time-varying model. Journal of System Simulation, 2011, 23(06): 1098−1103 [52] Zhao Zhong-Yuan, Li Nan, Ma Qin-Yong, Huang Jian-Ming, Wang Niu. Application of human simulation intelligent control method in acceleration control of DC motor speed regulating system. Journal of Central South University (Science and Technology), 2013, 44: 63−68 [53] Xu Peng, Xiao Jian, Li Shan, Yang Yi. Human simulated intelligent control for permanent magnet synchronous motor. Applied Mechanics and Materials, 2013, 241: 1821−1827 [54] 李锐, 郑太雄, 李银国, 冯辉宗, 陈伟民. 汽车防抱死制动系统分级智能控制. 机械工程学报, 2007, 08: 135−141 doi: 10.3321/j.issn:0577-6686.2007.03.023Li Rui, Zheng Tai-Xiong, Li Yin-Guo, Feng Hui-Zong, Chen Wei-Min. Hierarchical intelligent control of automobile anti-lock braking system. Journal of Mechanical Engineering, 2007, 08: 135−141 doi: 10.3321/j.issn:0577-6686.2007.03.023 [55] 涂亚庆, 陈浩, 杨辉跃. 一种自动泊车的仿人智能控制方法. 控制工程, 2014, 21(02): 161−167 doi: 10.3969/j.issn.1671-7848.2014.02.003Tu Ya-Qing, Yang Hao, Yang Hui-Yue. Autonomous parking method based on human-simulated intelligent control. Control Engineering of China, 2014, 21(02): 161−167 doi: 10.3969/j.issn.1671-7848.2014.02.003 [56] Zhang Wei-Zhong, Chen Gang, Hu Li-Ming, Wang Tao, Cui Shi-Tang, Jiang Qiang. Research of vehicle trajectory tracking based on human-simulated intelligent control. Advanced Materials Research, 2014, 940: 375−379 doi: 10.4028/www.scientific.net/AMR.940.375 [57] 王少杰, 侯亮, 黄鹤艇, 祝青园. 基于最优换挡控制目标的仿人智能模糊控制策略. 厦门大学学报(自然科学版), 2016, 55(01): 131−136Wang Shao-Jie, Hou Liang, Huang He-Ting, Zhu Qing-Yuan. Applications of optimal shift control strategy to human intelligent fuzzy control. Journal of Xiamen University (Natural Science), 2016, 55(01): 131−136 [58] Li Rui, Zhou Hong-Li, Li Xi, Zhang Ze, Wang Xiao-Jie. Human simulated intelligent control on magneto-rheological vibration isolation system for subway floating slab track with moving load. In: Proceedings of Intelligent Control & Automation. Guilin, China: IEEE, 2016.971−976. [59] Hua Yi-Ding, Gong Jin-Feng, Rong Hui, Wang Wen-Yang, Guo Peng, He Jia. Intelligent vehicle human-simulated steering characteristics access and control strategy. Journal of Shanghai Jiaotong University (Science), 2018, 23(1): 117−123 [60] 苏杰, 孙金龙, 刘友宽, 刘适. 基于仿人智能控制的火电厂AVC系优化研究. 电力系统保护与控制, 2018, 02: 157−162 doi: 10.7667/PSPC170442Su Jie, Sun Jin-Long, Liu You-Kuan, Liu Shi. Research on AVC system optimization of power plant based on human-simulated intelligent control. Power System Protection and Control, 2018, 02: 157−162 doi: 10.7667/PSPC170442 [61] Chen Zhong, Yan Wei, Xu Gao-Yu, Wang Guan-Jie. The UPFC intelligent control system based on human-simulated intelligent control. In: Proceedings of International Conference on Machine Learning and Cybernetics, Xian, China: IEEE, 2003.736-740. [62] Liu Li-Jun, Gao Hong-Ming, Zhang Guang-Jun, Wu Lin. Investigation on human-simulation intelligent control of the touch force in remote welding teleteaching. International Journal of Innovative Computing, Information and Control, 2006, 30: 85−88 [63] Zhang Kai-Sheng, Liu Jin-Hao. Study on human-simulated intelligent control method of fruit & vegetable cold satorage. In: Proceedings of IEEE International Conference on Computer Science & Information Technology. Beijing, China: IEEE, 2009.30−33. [64] 李国昉, 李栋, 齐玉斌, 毛志怀. 连续流动式粮食干燥机的仿人智能控制器. 农业工程学报, 2006, 07: 105−107 doi: 10.3321/j.issn:1002-6819.2006.10.022Li Guo-Zhen, Li Dong, Qi Yu-Bin, Mao Zhi-Huai. Human simulated intelligent controller for continuous-flow grain dryer. Transactions of the Chinese Society of Agricultural Engineering, 2006, 07: 105−107 doi: 10.3321/j.issn:1002-6819.2006.10.022 [65] 李国昉, 齐玉斌, 李栋, 毛志怀. 谷物干燥仿人智能控制系统. 农业机械学报, 2007, 01: 83−86 doi: 10.3969/j.issn.1000-1298.2007.01.021Li Guo-Zhen, Qi Yu-Bin, Li Dong, Mao Zhi-Huai. Human simulated intelligent control system for grain drying. Transactions of the Chinese Society for Agricultural Machinery, 2007, 01: 83−86 doi: 10.3969/j.issn.1000-1298.2007.01.021 [66] Xu Peng, Dan Yuan-Hong. Human simulated intelligent control of magnetic levitation systems. In: Proceedings of World Congress on Intelligent Control and Automation. Jinan, China: IEEE, 2010.1376−1381. [67] 杨辉跃, 涂亚庆, 毛育文. 科氏质量流量计振动幅值的仿人智能控制方法. 仪器仪表学报, 2019, 40(05): 118−123Yang Hui-Yue, Tu Ya-Qing, Mao Yu-Wen. Human-simulated intelligent control method for vibration amplitude of Coriolis mass flowmeter. Chinese Journal of Scientific Instrument, 2019, 40(05): 118−123 [68] Liu Yu-Cheng, Liu Yu-Bin. Human simulated intelligent control technique for incineration treatment of municipal solid waste. Information Technology Journal, 2013, 12(23): 7758−7761 doi: 10.3923/itj.2013.7758.7761 [69] 肖前军, 许虎. 生活垃圾焚烧炉燃烧过程温度的仿人智能控制. 智能系统学报, 2015, 06: 881−885Xiao Qian-Jun, Xu Hu. Human simulated intelligent control of temperature in the combustion process of domestic waste incinerator. CAAI Transactions on Intelligent Systems, 2015, 06: 881−885 [70] 敖朝华, 邓薪莉, 郭兵. 垃圾焚烧炉燃烧过程的优化控制策略. 环境工程, 2010, 01: 77−80+72Ao Chao-Hua, Deng Xian-Li, Guo Bing. Optimal control strategy of combustion process of waste incinerator. Environmental Engineering, 2010, 01: 77−80+72 [71] 李会军. 城市生活垃圾焚烧的智能控制策略. 重庆理工大学学报(自然科学), 2019, 33(01): 64−68Li Hui-Jun. Application research on intelligent control strategy for municipal solid waste incineration. Journal of Chongqing University of Technology(Natural Science), 2019, 33(01): 64−68 [72] 曾令辉, 曾建成. 酶解胎盘粉制备羊胎素粉剂量产工艺仿人控制实验研究. 制造业自动化, 2019, 41(01): 99−103 doi: 10.3969/j.issn.1009-0134.2019.01.022Zeng Ling-Hui, Zeng Jian-Cheng. Human simulated intelligent control experimental investigations of mass production process in prepar. Manufacturing Automation, 2019, 41(01): 99−103 doi: 10.3969/j.issn.1009-0134.2019.01.022 [73] 黄伟. 工业燃气窑炉炉膛温度的仿人智能控制策略. 重庆理工大学学报(自然科学), 2019, 06: 117−121Huang Wei. Control strategy based on human simulated intelligence in combustion chamber temperature of industrial gas kilns. Journal of Chongqing University of Technology (Natural Science), 2019, 06: 117−121 [74] 何成, 王耀南, 邹伟生, 成晓明. 矿浆管道输送流速仿人智能多模态控制研究. 控制与决策, 2012, 02: 295−298+303He Cheng, Wang Yao-Nan, Zou Wei-Sheng, Cheng Xiao-Ming. Research on human simulated intelligent multi-modal control of flow velocity of slurry pipeline transportation. Control and Decision, 2012, 02: 295−298+303 [75] 冯茜, 王磊. 仿人智能协调控制在循环流化床锅炉燃烧控制系统中的应用. 计算机测量与控制, 2014, 10: 3158−3160 doi: 10.3969/j.issn.1671-4598.2014.10.024Feng Qian, Wang Lei. Application of human simulated intelligent coordinated control in combustion control system of circulating fluidized bed boiler. Computer Measurement & Control, 2014, 10: 3158−3160 doi: 10.3969/j.issn.1671-4598.2014.10.024 [76] 雷正桥, 李雷. 燃气辊道窑炉燃烧过程的强鲁棒性控制. 自动化仪表, 2014, 12: 75−78 doi: 10.3969/j.issn.1000-0380.2014.04.022Lei Zheng-Qiao, Li Lei. Strong robustness control of combustion process of gas roller kiln. Process Automation Instrumentation, 2014, 12: 75−78 doi: 10.3969/j.issn.1000-0380.2014.04.022 [77] 胡洁. 基于仿人智能控制的无人船自动驾驶系统的研究. 舰船科学技术, 2017, 14: 46−48 doi: 10.3404/j.issn.1672-7619.2017.03.009Hu Jie. Research on unmanned autopilot system based on human simulated intelligent control. Ship Science and Technology, 2017, 14: 46−48 doi: 10.3404/j.issn.1672-7619.2017.03.009 [78] 唐月夏, 李光平. 货船甲板起货装置机电交流恒功率控制算法. 舰船科学技术, 2018, 22: 199−201Tang Yue-Xia, Li Guang-Ping. Electromechanical AC constant power control algorithm for cargo ship deck lifting device. Ship Science and Technology, 2018, 22: 199−201 [79] Li Zu-Shu, Wang Gui-Ping. Schema theory and human simulated intelligent control. In: Proceedings of IEEE International Conference on Robotics, Intelligent Systems and Signal Processing. Changsha, China: IEEE, 2003.524−530. [80] Li Zu-Shu, Zhang Hua, Wen Yong-Ling, Wang Gui-Ping. Human simulated intelligent control based on sensory-motor intelligent schemas. In: Proceedings of Intelligent Control and Automation. Hangzhou, China: IEEE, 2004.2423−2427. [81] 李祖枢. 仿人智能控制理论和多级倒立摆的摆起控制. 人工智能:回顾与展望. 北京: 北京科学出版社, 2006. 174−207Li Zu-Shu. Human simulated intelligent control theory and swing control of multi-stage inverted pendulum. Artificial Intelligence: Retrospect and Prospect. Beijing: Science Press, 2006.174−207. [82] 张华. 基于动觉智能图式的多级摆系统仿人智能运动控制[博士学位论文], 重庆大学, 2006Zhang Hua. Research of multi-pendulum system human simulated intelligence motion control based on sensory-motor schema[Ph. D. dissertation]. Chongqing University, 2006. [83] 吴恭兴, 万磊, 邹劲, 庄佳园, 廖煜雷. 混合仿人智能图式的无人艇自主驾控系统设计. 控制理论与应用, 2010, 27(12): 1647−1654Wu Gong-Xing, Wan Lei, Zou Jin, Zhuang Jia-Yuan, Liao Yu-Hua. Design of the autonomous pilot system for the unmanned surface vehicle based on the hybrid human simulated intelligent schema. Control Theory & Applications, 2010, 27(12): 1647−1654 [84] 但远宏. 二级倒立摆平衡点间任意转换的运动控制方法研究[博士学位论文], 重庆大学, 2017.Dan Yuan Hong. Study on arbitrary transfer movement control between rod’s equilibrium statuses for a double inverted pendulum[Ph. D. dissertation], Chongqing University, 2017. [85] Dong Xiao-Min, Yu Miao, Liao Chang-Rong, Chen Wei-Min. Human-simulated intelligent control of vehicle suspension with magneto-rheological dampers. Journal of Advanced Science, 2006, 18(1& 2): 103−106 [86] Tsai J T, Liu T K, Chou J H. Hybrid taguchi-genetic algorithm for global numerical optimization. IEEE Transactions on Evolutionary Computation, 2004, 8(4): 365−377 doi: 10.1109/TEVC.2004.826895 [87] Liu Tung-Kuan, Shen Yao-Chun, Li Zu-Shu. An application of multiobjective optimization genetic algorithm for cart-double-pendulum system control. In: Proceedings of IEEE International Conference on Systems, Man and Cybernetics, Taipei, China: IEEE, 2006.3292−3297. [88] Liu Tung-Kuan, Chen Chiu-Hung, Li Zu-Shu, Chou Jyh-Horng. Method of inequalities-based multiobjective genetic algorithm for optimizing a cart-double-pendulum system. International Journal of Automation and Computing, 2009, 6(1): 29−37 doi: 10.1007/s11633-009-0029-3 [89] 李祖枢, 但远宏, 张小川, 肖琳, 谭智. 双摆机器人摆杆平衡态任意转换运动控制的实现. 自动化学报, 2010, 36(12): 1720−1731Li Zu-Shu, Dan Yuan-Hong, Zhang Xiao-Chuan, Xiao Lin, Tan Zhi. Fulfillment of arbitrary movement transfer control between equilibrium states for a double pendulum robot. Acta Automatica Sinica, 2010, 36(12): 1720−1731 [90] Li Zu-Shu, Tan Zhi, Wang Yu-Xin, Zhang Hua. Real time swing-up and handstand-control of cart-double-pendulum system on an incline rail. In: Proceedings of China Automation Society Control Theory Committee. Wuxi, China: IEEE, 2004.835−839. [91] 李祖枢, 但远宏, 温永玲, 张华. 小车三级摆摆起倒立的仿人智能控制. 华中科技大学学报(自然科学版), 2004, S1: 38−41Li Zu-Shu, Dan Yuan-Hong, Wen Yong-Ling, Zhang Hua. Swing-up and handstand control of cart-triple-pendulum system based on human simulated intelligent control theory. Journal of Huazhong University of Science and Technology (Natural Science Edition), 2004, S1: 38−41 [92] 吴垣甫. 小车单摆系统仿人智能控制与参数优化仿真. 信息与控制, 2010, 01: 120−128 doi: 10.3969/j.issn.1002-0411.2010.01.021Wu Yuan-Fu. Simulation of human simulated intelligent control and parameter optimization of a single pendulum system for a car. Information and control, 2010, 01: 120−128 doi: 10.3969/j.issn.1002-0411.2010.01.021 [93] Xiao Lin, Li Zu-Shu, Dan Yuan-Hong. The up-down giant-circle movement control of cart-double pendulum using HSIC. In: Proceedings of Intelligent Control & Automation. Jinan, China: IEEE, 2010.1076−1081. [94] Chen Gui-Qiang, Wu Peng, Zhao You-Hua. Research of DD-DU for the cart-double pendulum based on human-simulated intelligent control. In: Proceedings of Intelligent Control & Automation. Shenyang, China: IEEE, 2015.3844−3847. [95] 王牛, 李祖枢. 一种两轮轮式机器人点镇定智能控制实现. 控制理论与应用, 2010, 27(04): 437−443Wang Niu, Li Zu-Shu. Realization of point stabilization intelligence control for two-wheel robot. Control Theory & Applications, 2010, 27(04): 437−443 [96] 刘成军, 薛方正, 李祖枢, 邓航见. 双足机器人动态步行仿人智能控制. 重庆大学学报, 2013, 36(02): 45−50Liu Cheng-Jun, Xue Fang-Zheng, Li Zu-Shu, Deng Hang-Jian. Human simulated intelligent control for dynamic walking of biped robot. Journal of Chongqing University, 2013, 36(02): 45−50 [97] 但远宏, 杨武, 唐继强, 谭智, 张小川. 双摆机器人倒立点到悬垂点的欠驱动控制研究. 机床与液压, 2019, 15: 29−32+37 doi: 10.3969/j.issn.1001-3881.2019.08.007Dan Yuan-Hong, Yang Wu, Tang Ji-Qiang, Tan Zhi, Zhang Xiao-Chuan. Research on underactuated control of double pendulum robot's inverted to overhang point. Machine Tool & Hydraulics, 2019, 15: 29−32+37 doi: 10.3969/j.issn.1001-3881.2019.08.007 [98] Dong Xiao-Min, Yu Miao, Li Zu-Shu, Liao Chang-Rong, Chen Wei-Min. Neural network compensation of semi-active control for magneto-rheological suspension with time delay uncertainty. Smart Materials and Structures, 2009, 18(1): 1−14 [99] Dong Xiao-Min. Semi-active control of magneto-rheological variable stiffness and damping seat suspension with human-body model. International Journal of Vehicle Design, 2013, 63(2/3): 119 doi: 10.1504/IJVD.2013.056098 [100] Dong Xiao-Min, Xiong G M. Vibration attenuation of magneto rheological landing gear system with human simulated intelligent control. Mathematical Problems in Engineering, 2013, 14: 1−13 -
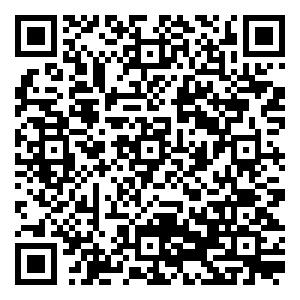
计量
- 文章访问数: 1455
- HTML全文浏览量: 474
- 被引次数: 0