基于非线性时序模型的神经网络图形识别方法
Nonlinear Time Series Model for Shape Recognition Using Neural Networks
-
摘要: 提出了复数域非线性指数自回归(CNEAR)图形轮廓建模方法.构造了用于CNEAR 模型参数估计的神经网络模型,利用该网络可解决非线性参数的估计问题.CNEAR模 型参数具有平移、旋转和比例不变性并与计算起始点的选择无关.基于模型参数构造了特征 向量,并设计了复数神经网络分类器,给出了复数神经网络学习算法.实验结果表明CNEAR 模型在较低阶次即能获得较高的识别率,CNEAR模型对带噪声图形及形状差别较小图形的 识别效果要好于复数域自回归模型方法.
-
关键词:
- 图形识别 /
- 复数域非线性指数自回归模型 /
- 神经网络
Abstract: A complex nonlinear exponential autoregressive (CNEAR)modeling approach for the closed shape boundary is suggested in this paper. A neural network structure is constructed to estimate the parameters of the CNEAR model. The nonlinear coefficient is easy to be determined by the network. The CNEAR parameters are invariant to translation, rotation around the origin, scale and choice of the starting point in tracing a boundary. The feature vector can be constituted by the CNEAR parameters. A complex neural network classifier is designed for learning and classification and its learning algorithm is also given. Experimental results show that high recognition rates can be achieved even for low-order models. It is also seen that the CNEAR model performs better than the complex autoregressive (CAR) model when shapes have random noise on the boundaries or have differentiating features at detailed levels. -
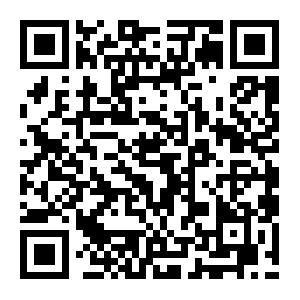
计量
- 文章访问数: 3297
- HTML全文浏览量: 97
- PDF下载量: 1223
- 被引次数: 0