Property Analysis of Hyperball CMAC and Multiple CMAC Structure
-
摘要: 如何选择合适网络参数是传统CMAC(Cerebellar Model Articulation Controller)应用 中的一个难题.采用泛化均方差(GMSE)和学习均方差(LMSE)来分别评价超闭球CMAC的泛 化能力与记忆精度,并引入权调整率的概念,来研究CMAC结构参数与学习性能的关系.研究 结果表明,在样本分布和量化级数不变时,泛化均方差和学习均方差是权调整率的非增函数.因 此超闭球CMAC在满足存储空间和计算速度的要求下尽量使得权调整率较大.还提出了并行 CMAC结构以进一步提高单个超闭球CMAC的非线性逼近能力.仿真结果证明了该方法的有 效性.Abstract: It is a problem to select network parameters of conventional CMAC. Generalization mean squared error (GMSE) and learning mean squared error (LMSE) are used to evaluate generalization ability and learning accuracy of hyperball CMAC. Weight adjusting ratio is introduced and investigated for discovering the relationship between the network parameters and learning property. Research results indicate that generalization ability and learning accuracy are the non-decreasing function of weight adjusting ratio. Therefore, the weight adjusting ratio of hyperball CMAC is chosen as large as possible with proper memory space and learning speed. A parallel CMAC structure is also designed for improving nonlinear approximation capabilities of the single hyperball CMAC. Simulation results demonstrate that the proposed strategies are very effective.
-
Key words:
- CMAC /
- neural network /
- generalization ability /
- learning accuracy
-
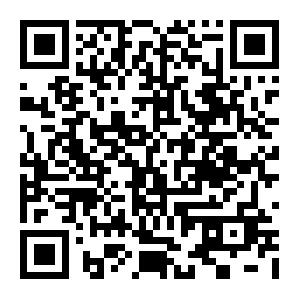
计量
- 文章访问数: 2047
- HTML全文浏览量: 78
- PDF下载量: 838
- 被引次数: 0